You have /5 articles left.
Sign up for a free account or log in.
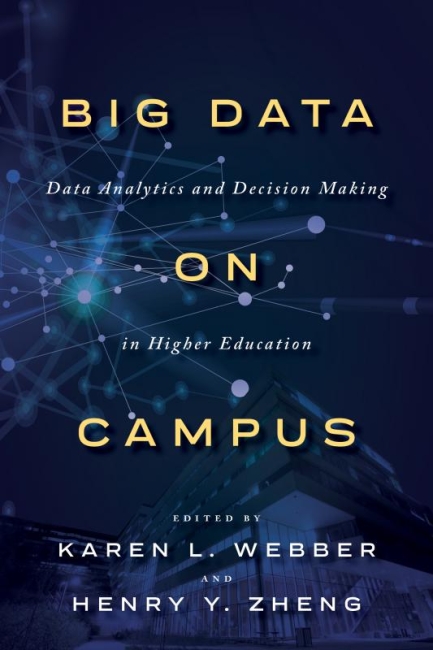
Johns Hopkins University Press
Amid the periodic enthusiasm for data’s ability to revolutionize the way colleges and universities work, it can be easy to forget that having more data available doesn’t necessarily translate into leaders making better decisions on campus.
It’s a point Karen L. Webber and Henry Y. Zheng make early in their new book, Big Data on Campus (Johns Hopkins University Press).
“Despite some newfound emphasis on data analytics, most higher education officials are not yet adept at using analytics to support institutional decision making,” they write.
The book is targeted at administrators, institutional researchers, technology workers and graduate students. But it’s a useful primer for anyone seeking to understand how higher education leaders can use the large amounts of data that are available on campuses today -- as well as how many are coming up short, plus a host of concerning issues surrounding the appropriate use of data.
Chapters cover topics ranging from principles of good practice to why data analytics can inform admissions decisions to decision making itself. Webber, a professor of higher education at the University of Georgia, and Zheng, senior associate vice president for strategic analytics at Ohio State University, edited the book and contribute in several of its chapters. The result is a range of case studies, examples and checklists for those who want to look around them and see how data can be harnessed at their own institutions.
Webber and Zheng emphasize several elements that need to be in place before smart data-informed decision making is possible on campuses: people, technology, process and culture. One of the key lessons to remember from a book about data is that the human element can’t be ignored.
“Along with more data comes the need to use contextualized knowledge of the higher education organization and analytics strategies that account for the unique situation or population under study, and everyone must be mindful of privacy, ethical, and overall responsible use of the data,” Webber and Zhen write.
The two editors combined to answer questions about the book via email. Their responses, which follow, have been edited lightly for style and clarity.
Q: Do you want everyone who reads the book to walk away with one or two key ideas?
A: Gosh, it’s hard to pick just two. It’s exciting to see the application of data analytics growing in higher education, and we are buoyed by the ways in which some of the techniques related to data analytics can improve student, faculty/staff and organizational success. If we had to choose:
- The ways in which we’ve thought about the use of data in higher education decision making [have] evolved over the past couple of decades. There’s an important distinction between data-informed and data-driven decision making. Whereas data-driven decision making (DDDM) lets the data speak for itself, data-informed decision making (DIDM) considers the context in which the decision is being made. We believe that analytic results are best when the information is examined within the specific situation or context, and that means that in some cases, the algorithmically generated answer or prediction with the highest accuracy rate may not be the best answer for the situation at hand.
- The use of data analytics to build strong and long-lasting data-Informed decision making requires certain kinds of people, technology and processes. It requires senior leaders who have the vision for data-informed decisions as well as knowledgeable and skilled data analysts, software engineers, data visualization specialists and staff who can communicate the findings. It requires devoting capital to a strong infrastructure of advanced technology, and it requires a coordinated campus plan that values the use of data (that has structures in place to ensure appropriate data use and is mindful of security and responsible use).
- We have to add a third (although not new to our book). Data is only helpful when it is transformed into useful information. Acknowledging the context is essential.
Q: Early in the book, you discuss how organizations can be data rich but information poor -- and how they don’t always know how to turn large amounts of data into action. Can you suggest any steps to help college leaders make the jump?
A: Indeed, collecting large volumes and varieties of data [is] possible today; storage in a campus-based architecture or the cloud is relatively easy and less expensive than in the past. This tempts us to collect data for the sake of collecting without having a thoughtful plan for how, when and for what purpose it will be used. An important part of a strong and comprehensive data governance plan includes identifying which data will be used to address certain institutional questions. Start by refining the questions that relate to institutional goals and then identify relevant data that will help to answer those questions.
Q: You outline elements that affect data-informed decision culture in higher ed: people, technology, process and culture. Is one more important than the others, or does one need to be in place first?
A: For optimal and effective implementation, all … are needed. Perhaps, though, it starts with leaders who have a strong vision. These leaders have the ability to apportion resources for the skilled staff (or ensure training to get staff the right set of skills), who can then identify the complement of technology needed. Leaders with vision initiate the processes because they realize that actions build culture. Leaders with a vision also realize that not all activities will be perfect, so they understand and allow for bumps along the way, knowing that building a data-informed culture doesn’t happen overnight and requires multiple efforts from many angles.
Q: As technology makes more information readily available for longer periods of time, tension exists between users who want to control their own data -- their own stories about themselves -- and organizations that engage in mass collection of data. If that tension continues, does it have implications for how colleges and universities need to approach big data?
A: Yes! This is such a critical question, thank you for asking. In today’s higher education world with the volumes and richness of data, no one unit can easily (nor perhaps should) handle all data requests. It is reasonable and appropriate to have select data users in a variety of offices on campus (such as admissions, financial aid, advising, HR, academic dean’s offices), however, it is critical that users receive thorough training on the definitions of the data and any contextual facets that are possible. In most cases, working with other senior colleagues on campus, knowledgeable leaders of institutional research offices should remain central in establishing user training, select data sharing, and ensuring data security through a strong and comprehensive data governance plan.
Q: Could predictive analytics represent an identity crisis for a sector that considers itself a driving force for opportunity and social mobility?
A: The nature of predictive analytics requires the use of previous data to make predictions about the future. Before a predictive analysis can be done, the analyst must consider if and how the previous data will introduce bias. If we’re interested in diversifying the kind of students in higher education with students that were not represented in previous cohorts, then the use of predictive analytics may not help us achieve the goals of greater access for underrepresented students.
Q: It seems that using data well requires a large number of resources, both staffing resources and financial resources. Do you have any ideas on how small institutions with limited resources might be able to overcome this fact?
A: Data-informed decision making is a key to effective institutional management. Start small; prioritize tasks or areas of focus that align with the institution’s mission. Finding small successes and building a culture of data-informed decision making will help each institution, large or small, move forward to better decisions.
Q: Was there anything you wanted to put in the book you couldn’t fit?
A: A few things:
- We intentionally kept the focus primarily on U.S. institutions. However, we acknowledge there are a number of institutions across the world that are ahead of the U.S. in their initial use of some aspects of data analytics in higher education learning and administration, in particular learning analytics.
- Many other examples of data analytics are available, and we learn of more each week. We already have some and can’t wait to gather more case examples to share. We’re also eager to get a better idea on which ones are yielding the greatest benefits. What works for one institution may not work as well at another. That may relate to the student population or the culture (and the value seen for data-informed decisions) or both.
- The use of learning analytics that capture multiple data points about an individual student to gauge performance and success, broadly, represents a fundamental shift in the use of data for student success. Prior models typically reported data in the aggregate and were careful to not provide information about individual students. However, many of today’s early-alert warning systems seek to gather a variety of data points for student performance at the individual level, often so that faculty members or advisers can help students improve before the end of a term. While the potentials of these alert systems can indeed help, they also have the potential to label individuals which can lead to unintended (negative) outcomes. It’s a fine line to walk. (We are not the first to acknowledge this change, but it’s a point well taken.)
Q: What did I miss in these questions?
A: Although the current challenges with the COVID-19 pandemic have forced us to shift our focus to immediate needs, we hope that we don’t lose sight of the larger goals and purposes of higher education that include developing a cultured, creative, racially and gender diverse population filled with students who will become our nation’s future leaders, thinkers, entrepreneurs and builders of the economy. How can we in higher education ensure our continued movement forward? Data analytics holds great promise for many areas within higher education and now more than ever, the use of data analytics to make data-informed decision is an important key to our future.