You have /5 articles left.
Sign up for a free account or log in.
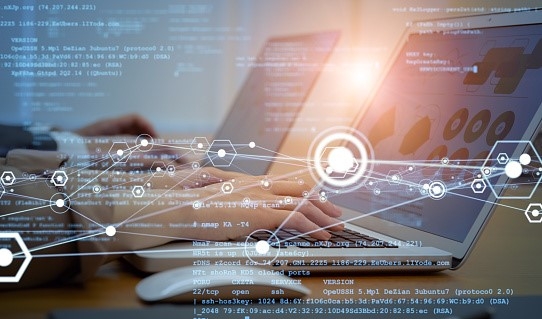
metamorworks/Getty Images
An algorithm is a process to follow in solving a problem, often in a mathematical calculation. Most people think of algorithms (if they think of them at all) as involving complicated formulas and computers.
In higher education, hundreds of colleges use algorithms in the admissions process. Their primary use is predicting how much money a student will need from the college to enroll. Colleges don't tell students they ran their information through a computer program, but they tell students what their aid package looks like. The same student will get a different package at different colleges. Only a minority of colleges pledge to meet students' demonstrated need. And many colleges -- public and private -- award aid that's not intended to focus on the lowest-income students.
Algorithms make the process more precise and more effective for many colleges. But do they serve students who need aid to succeed in higher education?
"Unfortunately, the widespread use of enrollment management algorithms may also be hurting students, especially due to their narrow focus on enrollment," said Alex Engler, a fellow at the Brookings Institution, in a paper this month. "The prevailing evidence suggests that these algorithms generally reduce the amount of scholarship funding offered to students. Further, algorithms excel at identifying a student’s exact willingness to pay, meaning they may drive enrollment while also reducing students’ chances to persist and graduate."
Engler added, "Higher education is already suffering from low graduation rates, high student debt, and stagnant inequality for racial minorities -- crises that enrollment algorithms may be making worse."
The paper, published by Brookings, has attracted many readers. Colleges don't want to talk about it. There are other observers of higher education who have pointed to the use of algorithms as a potential way to minimize the enrollment of low-income and minority students. They are -- not surprisingly -- cheering the paper. And vendors are strongly disputing the paper.
Set of Reforms
Engler not only criticizes colleges for using algorithms, he has a set of reforms to propose.
- "Categorically, colleges should not use predicted likelihood to enroll in either the admissions process or in awarding need-based aid -- these determinations should only be made based on the applicant’s merit and financial circumstances, respectively.
- "When colleges do use algorithms to distribute scholarships, they should proceed cautiously and document their data, processes, and goals.
- "Colleges should also examine how scholarship changes affect students’ likelihood to graduate, or whether they may deepen inequities between student populations.
- "Colleges should also ensure an active role for humans in these processes, such as exclusively using people to evaluate application quality and hiring internal data scientists who can challenge algorithmic specifications.
- "State policy makers should consider the expanding role of these algorithms too, and should try to create more transparency about their use in public institutions.
- "More broadly, policy makers should consider enrollment management algorithms as a concerning symptom of pre-existing trends towards higher tuition, more debt, and reduced accessibility in higher education."
The role of algorithms in admissions and financial aid, Engler asserts, is in awarding aid designed to convince an admitted applicant to enroll even if perhaps the student isn't getting enough money.
“This is precisely where algorithms shine -- they help financial aid offices make better educated guesses about how scholarship funding increases likelihood of student enrollment,” Engler wrote. “In one academic study, this resulted in a 23.3 percent increase in enrollment yield (from 12.1 percent to 14.8 percent) of out-of-state students. One major vendor advertises a ‘10 percent increase in enrollment’ through scholarship optimization.” (The vendor is EAB. More on EAB’s views later in the story.)
The danger of algorithms, Engler said, comes after they are used to predict the size of an admitted class. Wrote Engler, "There is also a second critical step, which is the optimization of decisions that the college can make, especially around providing scholarships to accepted applicants. While optimizing scholarship disbursement has historically been done manually, there is an emerging trend of using optimization algorithms, which raise additional concerns."
In this part of the process, Engler wrote, algorithms "enable financial aid offices to design a strategy for how much funding each applicant will get. This strategy is then run through the predictive yield model to see how it is expected to affect enrollment. A strategy might be as simple as segmenting the students based on high school GPA and SAT scores, and then giving $5,000 to the bottom third of applicants, $10,000 to the middle third, and $15,000 to the top third. Based on conversations with vendors and software demonstrations, an applicant’s expected family financial contribution also plays a prominent role, in addition to their GPA and SAT scores. The proposed strategies can segment students by many other variables -- vendors mentioned specific high schools, distance traveled to attend, Pell Grant eligibility, and more. In emails and conversations, several vendors also described how their process can even allocate both a mix of need-based and merit-based aid."
Engler sees many dangers in this approach. "First, when these algorithms work as intended, they may reduce average per-student scholarship support," he wrote. "Second, it is problematic that these algorithms typically optimize scholarships for yielding students, rather than using scholarships to support student graduation and success. The third concern is the possibility of algorithmic bias, through which subgroups of applicants who appear to the algorithm to be less affected by changes in scholarship funding may be mistreated. While the data necessary to definitively confirm these problems is not public, the available evidence gives weight to these concerns. Given that so many American colleges are now applying enrollment management algorithms, their total impact is potentially dramatic, and thus they deserve careful consideration and further study."
In an interview, Engler said that the real danger of the algorithms is offering students "just enough money for a student to attend," which is less than it will take for a student to thrive. The process, he said, gives "a systematic advantage to the university" in negotiating the size of aid packages. He sees the vendors, not the colleges, as the group that is pushing colleges in the wrong way.
Support for the Charges
The article was endorsed by Iris Palmer, a senior adviser for higher education and workforce with New America, who has written about algorithms in the past.
She said that when colleges use algorithms, they are making choices to favor wealthier (and whiter) students. For example, she said a college can discover that students from certain areas are more likely to enroll.
"When you are targeting certain ZIP codes, you are targeting certain kinds of people," Palmer said.
Palmer said she doesn't think colleges think algorithms are a way to avoid racial discrimination charges, but they avoid thinking about the conclusions of what they are doing.
"When you have a system that is set up to maximize tuition revenue, you are just in a system," she added.
Other Perspectives
Of the vendors in the space, Ruffalo Noel Levitz declined to comment. (The article identifies RNL as having one of the largest algorithm businesses, with hundreds of colleges.)
An EAB principal, Nathan Mueller, responded for his company. "I disagree with the author’s assertion that the prevailing evidence shows algorithms generally reduce the amount of scholarship funding offered," Mueller said. "The use of such algorithms is more likely in practice to encourage increases in funding as a means to increase enrollment and net tuition revenue."
He also denied that the service could reduce retention. "The quality of a student’s academic preparation before college has a much stronger correlation to that student’s persistence than does financial aid," he said. "In cases where EAB analysis suggests a strong correlation at a given institution between aid and retention, we work with that institution to adjust the aid strategy to compensate. We do that because it benefits students and because it is more economical for schools to keep students on path to graduate than it is to allow a student to fail, drop out, and lose the associated tuition and other revenues."
And Mueller also said the algorithms do not hurt students. "To the extent that an institution’s incentives are to maximize new enrollment and increase net revenue without regard to diversity or equity, they will -- lacking information to prove the contrary -- tend to cling to erroneous assumptions: that high-need students always cost more or that giving a large number of generous scholarships will help attract more of the students they want. EAB’s optimization work can correct these myths. It has been my experience that institutions are mindful of the trade-offs associated with balancing their need to generate the tuition revenue they require to operate, with their commitment to improving diversity, equity and student persistence."
Andy Hannah, president of Othot, a Liaison company, said a key flaw in Engler's thinking was its limited view of the way algorithms are used. For instance, he said they are used when admitting students and giving aid awards for the first time, as Engler suggests. But his company's algorithms are also used after that, he said, and help colleges with retention.
"We look for indication where there might be issues," he said. "We look at grades, majors, how students do in class, and advise."
Robert J. Massa, principal and co-founder of Enrollment Intelligence Now, was previously in admissions at Drew University, Lafayette College, Dickinson College and Johns Hopkins University.
"Algorithms in and of themselves are neither good nor bad for college admissions," he said via email. "The key is, how are they used? It’s important to remember that predictive models will not help admissions officers to make decisions on individual students, whether for admission or for financial aid. They will assist enrollment managers in making decisions on groups of students with similar characteristics."
Massa added, "Algorithms, if used properly, can actually help to increase access. Colleges and universities need revenue in order to fulfill their mission, whether in the classroom or in the shape and composition of their enrollment. It is important to optimize revenue among students from families with income sufficient to pay most or all of the price charged. So-called merit scholarships are rarely awarded to reward student performance in high school. Rather, colleges discount their price in order to change a student’s enrollment decision from one college to their college.
"The dollar amounts of these discounts, or merit awards, are often times arbitrarily chosen based on a general sense of what peer institutions might be doing with their merit scholarships. Arguably, this is an inefficient way to discount price. To optimize revenue from those families with ability to pay, it becomes necessary to award not a dollar more or a dollar less than it would take to influence their enrollment decision. A model that helps to optimize revenue in this example will, in fact, increase funds available to needy students."