You have /5 articles left.
Sign up for a free account or log in.
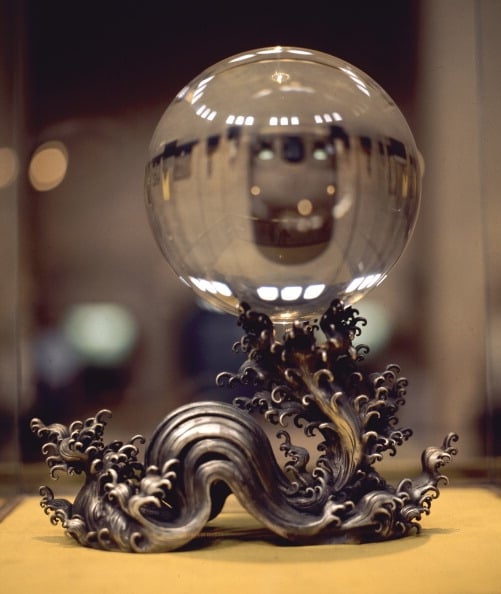
Getty Images
"If the information from predictive analytics could be discouraging, do we have a duty to withhold it? Is there an ethical basis for a sort of statistical placebo?"
That question closed a recent post by Matt Reed, author of "Confessions of a Community College Dean," an Inside Higher Ed blog. In the post, Reed discussed the issues related to "stereotype threat," and raised the concern that some uses of predictive analytics could result in unintentionally discouraging students whom colleges want to help.
The post received many thoughtful comments, and Inside Digital Learning posed the question to leaders of companies that work in predictive analytics or related fields. How would they answer? Many of them responded to our request for a short response, and we share their answers here, in alphabetical order by company.
Civitas Learning, from Mark Milliron, co-founder and chief learning officer:
If your family has a history of heart disease, we generally accept the notion that surfacing risks, like high cholesterol, provides advanced notice of something that could potentially be an issue, and ensure that you have the resources and support, including preventative care, to bend the curve on outcomes. In the same vein, we’ve found that using thoughtful design thinking to determine how to provide students with useful context, insight, and direction is critical.
However, transparency has to be anchored in a “do no harm” ethic. Data delivered the wrong way can be disastrous -- as any good classroom teacher would tell you. Indeed, when delivered with timely, thoughtful recommendations from administrators, faculty and advisers, or direct visualizations in apps, this right information can empower students to take more ownership of their pathways and life trajectories. This principle is fundamentally aligned with higher education’s developmental and democratic promise, and this is exactly how our partners approach using predictive analytics to better support their students.
EAB, from Melinda Salaman, director of strategic research, EAB Navigate:
Students have a right to know if predictive models exist at their institution and to see their own assessments if they wish. But where predictive analytics is a science, advising is an art. At EAB, we believe that adviser training built on best practice research is a necessary complement to predictive analytics. The human part of the equation cannot be underestimated. For example, the best practice research behind EAB Navigate surfaced a four-step plan for having difficult academic conversations with students. The key is to start with the positive by building a relationship with the student. Next, comment on an academic strength the student exhibited and inquire about their overall academic progress (“You have done really well in your psychology courses, but I notice you’re struggling in a few courses/subject areas”).
The adviser can then choose to share data that puts students’ performance into context (“At this college, students who get below a B- in this course are less likely to graduate in the major”). Lastly, advisers must offer themselves as thought partners to help students decide what to do with the information (“You will need to focus on the courses I highlighted moving forward if you want to stay in your major, or we can consider other options. Tell me more about the goal you’re working towards when you graduate.”). Empowering advisers with the expertise and tools they need to provide meaningful support -- and guiding students to make good decisions early on -- is key to promoting student success.
Ellucian, from Henry DeVries, principal consultant with a primary focus on analytics:
When I speak with business analytics and student success clients, I explain the activity of predictive analytics with the parallel question, “Based on what’s already happened, what do we think is going to happen next?” Matt Reed raises an interesting question when a predictive analytics model describes an outcome which could be discouraging regarding the academic success of a student -- “should one share or withhold that discouraging information?”
In my opinion, a faculty member or student adviser should absolutely share that information with the student, to withhold it could be intentionally misleading and may be more damaging in the long run. But, even when sharing the discouraging information which is triggered by a predictive analytics model, the faculty member or student adviser bears equal responsibility to share advice on the variety of appropriate activities (better class attendance, tutoring, other academic support) so that the student has an option to remediate the possible problem rather than to just do nothing.
Nevit Sandford’s model on Challenge and Support for student development also speaks to this scenario, I think. Sanford calls on faculty and staff to provide challenging opportunities (e.g., academic rigor) coupled with supportive structures for students (e.g., academic support resources) to promote student success in face of the challenge. Finally, it is important to remember the challenge of that potentially discouraging information cited by Reed arises from a predictive analytics model which may or may not fully match the characteristics of the specific student in question. Each student is unique and should be treated as an individual and the focus of advising and intervention should always be on the student’s actual performance and behavior, not just the composite predictive analytics model.
Helix, from Matthew Schnittman, president and CEO:
Any utilization of predictive analytics in education should be built on a fundamental desire to increase success for both the student and the institution. The notion of predictive analytics in education brings up understandable concerns, and mitigating those concerns becomes admittedly nuanced. Understanding a real risk profile requires a portfolio of data points -- socioeconomic indicators, work history, dependents, previous experiences with college, class schedule, etc. It should never be lost on anyone, however, that these points only come together to provide some confidence interval for what may happen, not what will happen. And the bottom line for the use of data analytics in education is that these confidence intervals should then inform an institution’s engagement strategy to help students navigate their options and their roadblocks to success. Only when coupled with high-touch, human interaction -- though academic advising, financial advising, program planning, and coaching -- can student-level predictions reach their highest expression for both the student and the institution.
Hobsons (including Starfish), from Ellen Wagner, vice president of research:
Answering the question starts with an understanding of why one would want to use predictive analytics in the first place, and then how to best apply the specific analytics to find answers to the question. Predictive analytics don’t result in a single correct answer emerging, a la Magic 8-Ball. It’s more about surfacing patterns and understanding what kind of variables can impact student success. Predictive analytics shouldn’t curtail students’ dreams or to send the message that we don’t think they can make it. Rather, they offer proactive prompts for institutions to find personally relevant pathways for success, surfacing risks that might be overlooked, or surfacing patterns that may not be intuitive. Analytics enable student success staff on campus to address concerns before they become problems, rather than communicating a message of anticipated failure.
InsideTrack, from Dave Jarrat, vice president:
For student support professionals, predicting a student’s likelihood of failure is neither straightforward nor particularly useful. There are an infinite number of factors at play and what really matters is what you are going to do to maximize the student’s chances at success. The right way to think about applying predictive analysis to issues of student success is to start with two basic questions – 1) what type of support is a student most likely to need? and 2) how likely is that student to engage with that support based on how it’s offered/delivered?
For example, a student who is working full time and raising children has a high likelihood of benefiting from time management support and a higher likelihood of engaging with that support if it’s offered in a convenient fashion. It’s not relevant to that student that, “XX% of working parents fail to persist.” It is relevant to them that, “students who watched these 2 short videos on balancing work, family and school obligations were XX% more successful and less stressed.” It’s not a matter of whether you should share predictive data with students or not, it’s a matter of sharing data they can act on.
Jenzabar, from Eileen Smith, vice president of marketing and communications:
At Jenzabar we position predictive analytics to our clients as a tool to assist in guiding students -- not as a tool to be utilized with a singular student or classroom of students in a negative manner. We encourage our clients to utilize predictive analytics in the analysis of how they can assist populations of students in being successful. For instance, Community College X knows that students from High School Y do much better in Math 101 if they receive remediation first -- the predictive analytics bear that out. With that knowledge, the institution can appropriately guide that cohort of students in their successful journey at their college. They don't need to begin their conversation with the student on a negative note, but instead illustrate their knowledge of the student and their dedication to making them successful. When used as an assistive tool, instead of a negative indicator, innovative institutions are able to see trends and act on them instead of waiting for an issue to arise.
Knewton, from Ryan Prichard, president and acting CEO of Knewton:
We see the positive power of predictive analytics borne out through adaptive learning. In fact, at Knewton, we see it as a useful tool that helps educators and institutions make informed decisions that support learning for all students. However, here’s the caution: students should never be limited in their development because of poor algorithms. As the community of adaptive learning providers grows, we must stay vigilant about building the most accurate and data-driven systems and products -- that is our responsibility to anyone we serve.
Additionally, content and courses alike should not be recommended on the basis of how well the student will do, but instead on the basis of what the student needs most to meet learning goals, what will help them stretch, and ultimately how each and every student can be given the opportunity to reach their highest potential. Using analytics to inform a student’s learning path moves away from using little or no data to lump students together, and instead toward an understanding of the unique abilities of each student. Being data-driven means that we’re not guessing at any point about the student’s knowledge and that we are applying the information we have to help them succeed at the highest rate possible. (Read our latest blog post from our Data Science team for more.)
How much is too much data for the student? This is a central question we are asking ourselves as we build and pilot adaptive courseware at institutions across North America. Data can certainly be a powerful motivator to know how you’re doing, what you’re excelling at, and what you need to know to move to the next level. In short, there are smart and measured ways of giving students insight into their own learning.
At a product level, we’ve identified an array of features that address this: suggesting study breaks if a student has been working nonstop, positively reinforcing hard work and strong performance, and providing encouragement when coursework is given that will likely to be tough for the student. It’s less about withholding certain data from the student, and more about being positive, constructive, and showing progression when presenting data to the student. One area we are still researching is how much data to provide in certain settings. For example, providing data to a student to compare themselves to other students in the class could be motivational for some students, while demotivating for others. At Knewton, we feel that factoring student mindset into the data equation is crucial for finding the correct balance of data to motivate each individual learner.
Macmillan, from Ken Michaels, chief executive officer:
Three points to make:
1) If the information from predictive analytics could be discouraging, do we have a duty to withhold it? Put another way, would one withhold health information because it may cause alarm? No, one would release it in the right context. If the risks are causing a social problem, that is an important problem to be solved (not a reason to withhold information). We should share.
2) We believe the big opportunity is how predictive analytics are presented, empathetically and in context that empowers action in supporting decision making. The point is surfacing and correcting by presenting "empathetically."
3) "Perfect" predictive algorithms are hard and so there are limitations. We need to better understand impacts on learners when a false alarm occurs (predicted at risk when they are not) and surprises (when a student is assessed as not at risk, but are). Algorithms attempt to balance these but future research is needed given the growing use of predictive analytics. Rigorous studies are needed to understand how risk assessments affect students depending on how those results are presented. More specifically, those with false alarms and less those with surprises as there are insights to be culled which is the spirit of Matt's message.
Pearson, from Angie McAllister, senior vice president for personalized learning and analytics:
The first thing to note is that predictive analytics is a bit of a misnomer; this is less about “prediction,” and far more about taking the right actions at the right time to drive student success. Predictive analytics draw on observed records of experience (aka “data”) and suggest, based on learner models, what state a learner might be in with respect to a desired outcome. So for example, an “early alert” or analytics insight should be put in front of the person that is best poised to take the action that will help the student move in the direction of success. Sometimes, that’s the student. Sometimes, it may be an instructor or student services or someone else that can support the student in a personalized way.
For effective teaching and learning, students and instructors need to know what records of experience -- or data -- are being collected, for what purposes, and how those data and insights are being shared and used. It’s also important to consider when designing analytics features in learning products that people may behave differently when they know they are being “observed.” Sometimes, students have motivational or affective reactions that impact learning, so we look to instructors to be the ultimate orchestrators of their classes, as they are the true key to successful individualized learning.
Taskstream, from Courtney Peagler, vice president of strategy and business development:
We do not offer predictive analytic capabilities -- nor do we claim expertise in this area. But, as a company that offers technology to help institutions gather, organize and use data to improve student learning and institutional quality, we are very interested in the types of questions Matt raises in his post. Part of the issue has to do with the type and level of granularity of the data on which the predictions are based, the level of the predictions being made, and what decisions are based on them.
For example, in Matt’s scenario of “steering students into the courses in which they’re statistically likeliest to succeed,” there is a greater risk of stereotype threat if the predictive model is based on broad demographics -- such as race, socioeconomic status, or gender identity -- and the measure of success is equally broad -- such as, the course grade or completion. If, however, the university were able to use more specific data for both the demographics and the measure of success, the resulting prediction might be more helpful and raise fewer ethical concerns.
That is, instead of saying, “students like you [i.e., similar demographics] tend to get a low grade in this course,” they could say things like, “students with similar written communication skills to you are more likely to get a low grade in this course” or “students who have taken these three courses before taking this course tend to demonstrate stronger written communication skills.” Although not perfect predictors for how well the student will be able to write after taking the course, at least the student can do something with the information (e.g., take the other courses first).
In higher education today, most of the data institutions have to be able to make predictions about student success aren’t particularly helpful when it comes to student learning. You may be able to predict if a student is going to “do well” in a course based on the number of times they do something in the LMS course, but that doesn’t tell you anything about what the student actually learned. If we can move to a system where judgments of learning aren’t discussed simply in terms of assignment/course grades, but rather in terms of specific learning outcomes demonstrated, perhaps the question of whether predictive analytics should be shared with students wouldn’t be quite so thorny.
VitalSource, from Michael S. Hale, vice president for education:
Matt Reed poses some interesting questions. I contend that we have a strong obligation to share information when we know that there are proven ways to combat the potential negative effects predicted for students that share a set of characteristic. In fact, the point of predictive analytics is to use the data to provide particular students with the tools, programs and support they need to be successful based on what we know has been successful with students with those characteristics. Predictive analytics are diagnostic and it would be educational malpractice if we had the knowledge of a successful strategy and/or support plan and did not deploy it.
Mr. Reed gives worries that applying predictive analytics could result in “inadvertently confirming negative stereotypes” by “steering students into the courses in which they’re statistically likeliest to succeed could easily mean recreating existing economic gaps, only with the blessing of science.” Given that the questions are the result of his chance encounter with Claude Steele, it is worth reviewing what actions Professor Steele took following his initial research identifying the “stereotype effect.”
Steele did almost the opposite of Reed scenario; he developed programs for students most likely to be negatively affected by the effect that put them into more challenging courses, not less challenging, and put them in study groups to bring social network support to counteract the effect. Since that time, many other effective supports and programs have been developed for very specific student profiles that have been provide to be successful. I would argue strongly that we are doing students a disservice by withholding information -- equivalent to a physician diagnosing a patient, identifying a treatment plan, then withholding the plan from the patient. In the medical world, that would be considered malpractice.
Of course, every student is an individual and there are limits to predictive analytics and recommended actions, just as there are limits to medical efficacy. The difference is that our educational models in higher education have been based on the assumptions that all students are the same.
Workday, from Liz Dietz, vice president for student strategy and product management:
The ultimate goal for higher education institutions is to successfully help students graduate and move into the workforce. Predictive analytics can be a powerful tool in helping institutions gain insights on student, faculty, and curriculum trends, which allows them to proactively identify areas of investment to help students succeed. Gartner has even noted that predictive analytics is a top 10 strategic technology for higher education, and is "a key part of strategies to improve student success and save money through improved retention."
Institutions are now laser focused on designing and executing on personalized student experiences to ensure they are setting students up for success. Too often, institutions are crippled by relying on reactive student support strategies. With predictive analytics, however, institutional leaders can proactively identify and inform student success teams of the students most likely to be at risk based on a wide variety of elements. By knowing how to leverage predictive models in the most meaningful way, institutions will be better able to achieve their goal of helping students move into the workforce.