You have /5 articles left.
Sign up for a free account or log in.
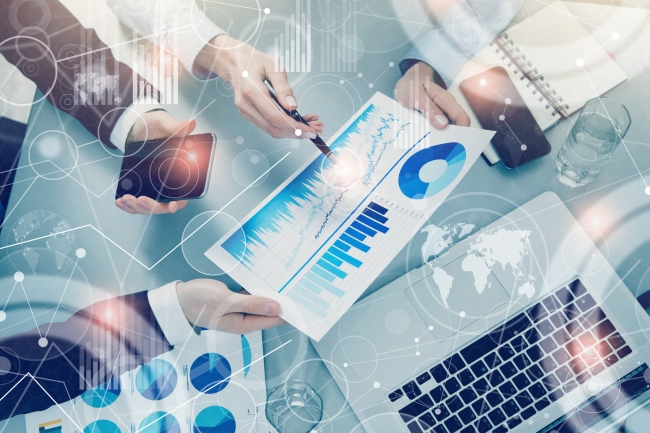
istockphoto.com/Erikona
If you ask, many people will say we are in a new era of higher education, one where machine learning and big data analytics are driving rapid change. From the influx of adaptive learning technologies to the automated student support services and predictive analytics models driving new interventions, there are fewer spaces of college and university life that are not being touched by these technological innovations.
These technological opportunities could offer a lot to higher education. Indeed, if we ignore the opportunities that machine learning and big data analytics might provide to complement our human capacities, we will do a disservice to those we claim to serve -- our students.
But if we treat them as an opportunity to downsize the work force or largely replace human social interactions with automated ones, we are going to lose a lot more than we gain. Herein lies the dilemma. What is the balance?
Given this time of turbulent change, I want to offer some reflection on the future of higher education in the age of machine learning and big data analytics. I do so in the context of what these technological opportunities may provide and how higher education has to manage its relationships to those technologies across three key areas: teaching and learning, predictive analytics, and student support.
In the age of machine learning and big data analytics, higher education is being offered the opportunity to personalize its education through adaptive learning technologies. As the machines get smarter, so too do the adaptive learning algorithms that can respond to students. This is not, of course, a wholesale swap-out of faculty-led teaching for machine-driven learning. It is way too early for that world.
But the adaptive learning movement -- driven by a growing for-profit educational technologies industry -- should produce a moment of pause for higher education. The promise underneath these technologies is the notion of a hyperindividualism whereby each person can collect up skills and credentials with less and less social interaction. In fact, adaptive learning technologies, while not being built right now to take the place of everyday teaching and learning, can feed into a prevailing mind-set that continues to call into question the value of higher education, the role of the university and college, and the teaching and learning that take place there.
If we have learned anything from the slew of reports that suggest that fewer and fewer adults see the “real value” of higher education, the ability to bypass the critical thinking work that goes on in the day-to-day world of the university becomes appealing to those who are ideologically opposed to a broad liberal understanding of higher education. Instead of adaptive learning playing the role of supplemental tool -- and a very good one in many cases -- these technologies could be sold off as a cheaper, faster and less contentious road toward a credential that will support advancement in the future work force.
What is lost by this move is the interstitial work that happens in universities that continue to bundle their education into programs that focus student attention on the interconnections, debates and opportunities provided by thinking across different topics, concepts and ideas.
Technological advancements are also shaping student success and the big data futures promised by predictive analytics. Committed to one of the core values of machine learning is the potential to analyze large sets of data that can be used to focus more intently on the individual learner. No longer will institutions be beholden to crude models that rely on population-level metrics for cohorts of students. Instead, variables can collide in a big data machine and focus attention on the individual student in a much more granular way.
There remains a lot of uncertainty in the big data futures that many companies and universities are promising. Even after the machine learning algorithms provide results, the question of why a certain finding appears can often remain a mystery; it still takes good old-fashioned qualitative work with faculty, staff and students to really understand the answer the machines might be giving us. Machine learning and big data analytics could also push universities to provide solutions to students that might not be in their best interest. Those solutions, if built on a deficit model instead of a growth mind-set approach, might track students into support services or academic enrichment programs that create a sense of isolation from instead of a sense of belonging to the wider campus community.
The caution here is that the machine learning algorithms can only do so much. The “noise” found in what the system is “not telling us” remains just as important as the predictions the algorithms might generate. Practitioners have to interpret the machine learning-based results. If not, universities are going to be driving their strategies toward machine learning-derived outcomes that might not impact student success.
Put another way, while big data analytics provide a real opportunity to move toward more predictive models and interventions, if universities de-invest (or not invest at all!) in the talent to interpret those results and act upon them, they will likely find little value in their long-term value proposition.
Higher education is seeing opportunity produced by machine learning and big data analytics in the space of directed student support. New technologies are emerging that allow students to map their progress toward degree, figure out their next academic step, connect to employers and even build skills that enhance their education along the way. There is real promise in this future, particularly if these technologies can help institutions scale support in ways that are not tenable in an environment where they have more students and those students are taking advantage of online and hybrid education to diversify their learning experience. Students are simply not in the same place at the same time anymore, and technological interventions can help recreate some of that social loss if managed effectively.
Perhaps, most importantly, machine learning and big data analytics provide higher education the opportunity to unfetter learning from the rote system of knowledge acquisition and take advantage of learning analytics to more deliberately engage students. The challenge of any such system, of course, is the question of to what are you building connections? Is it to the system or to each other? If it is to each other, what are the goals of making those connections and to whom? But a responsive system that can easily direct students to resources can go a long way in helping institutions manage the much more complex future of student learning, where students are not arriving in first-time, full-time cohorts to complete a degree 15 units at a time.
I believe there remains a lot of potential in a future of machine learning and big data analytics. But, for it to be realized, higher education may have to either commit to a certain level of privacy invasion -- students will have to volunteer more and more data to refine the models -- or sacrifice certain analytic power to provide students the relative privacy they want to maintain. How universities create systems to both protect privacy and support students will be a challenge as the privacy debate heats up both locally and globally.
Given this and the many other issues outlined above, I am neither an optimist or a pessimist when it comes to the future of higher education in the age of machine learning and big data analytics. I am, however, trying to be realistic and responsive to the potential futures that new education technologies present. In that response, I hope that universities pause and reflect on the equally important human capital and social interactions that remain essential to higher education and leverage real opportunities, such as those afforded by open educational resources or collaborative, student-centered virtual learning tools based in the principles of universal design, for example, to create a better learning future.
What this all suggests is that higher education should have a serious and ongoing conversation about how we place machine learning and big data analytics into our institutions and what infrastructures these new technologies demand so that they are responsive to us and not the other way around.