You have /5 articles left.
Sign up for a free account or log in.
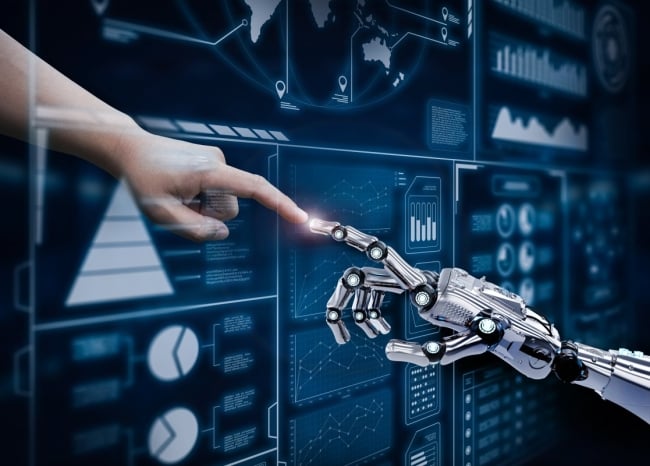
Istockphoto.com/PhonlamaiPhoto
Artificial intelligence, it seems, is infiltrating every corner of higher education. From improving the efficiency of sprinkler systems to supporting students with virtual teaching assistants, AI has quickly become a near-ubiquitous presence on some campuses.
Perhaps with good reason. Colleges and universities are being asked to do more with less as they grapple with shifting demographics and the need to not just respond to, but also anticipate, the needs of today’s students. And early returns suggest that AI can play a role in helping institutions tackle pernicious challenges -- from “summer melt” to student engagement -- and enable students to navigate the complexity of financial aid, admissions, campus life and course scheduling.
In response, a growing number of products are touting AI and machine learning as part of their sales pitch. But what’s the difference between AI that can actually help students succeed and so-called AI that is nothing more than decision trees or marketing ploys? How can institutional decision makers cut through the jargon to identify the sort of AI that can actually learn over time and improve outcomes?
College and university leaders are facing growing pressure to get smart on artificial intelligence. And that starts with a basic understanding of what AI actually is and how it works. Here’s a glossary of key AI terms designed to help higher education decision makers navigate the landscape.
Machine learning (ML): The process that enables systems to learn and improve from experience without being explicitly programmed. Machine learning generally refers to the development of computer programs that can access data and use them to learn and make predictions for themselves.
Why it matters: Everything we think of as AI starts with machine learning. A program’s ability to learn on its own is the foundation of artificial intelligence. That said, while all AI is machine learning, not all machine learning is AI.
Artificial intelligence (AI): Machine learning that resembles what we consider human intelligence. There are two broad categories: “narrow” AI (or ANI) is programmed to perform a single task, such as predicting the weather or playing chess. “General” AI (or AGI) can successfully perform any intellectual task that a human being can.
Why it matters: Currently, general AI only exists in the realm of science fiction (think Her or 2001: A Space Odyssey). That said, the landscape of narrow AI is becoming ever more sophisticated. We don’t have self-driving cars yet, but we do have Star Trek-like translation, personal assistants like Alexa and Siri, and increasingly precise credit card fraud detection. Narrow AI is invisibly making our lives significantly better, which is why we shouldn’t underestimate its potential in higher education.
Neural network: AI is based on the idea of neural networks -- essentially artificial brains built from silicon. These networks take a structural, layered approach to processing information, based on the way the human brain works: each layer of the network (made up of artificial “neurons”) provides the input for the next layer. Over time, these layers learn to recognize patterns that can, for example, differentiate a photo of a dog from a cat.
Why it matters: Neural networks are what enable AI to “learn” from user behavior. AI technologies typically start with very little information, but the neural network enables them to build expertise over time based on the data they take in. For instance, the more an AI receives questions from students about FAFSA completion details, the more quickly it will recognize how to respond to those questions.
Chat bot: The user interface for most computer programs is a mouse, keyboard or touch screen. Chat bots, like Siri and Alexa, provide a different kind of user interface for AI systems that uses speech (either spoken or typed). These AI programs range in sophistication from relatively simple and rule based (e.g., providing a canned response to a specific question) to more complex and AI-enabled programs, which can parse human language and learn from previous conversations to improve accuracy.
Why it matters: It is easy to build a simple chat bot but complex to build a genuine AI chat bot. (There’s a whole website about it.) A chat bot’s ability to understand run-on questions, handle misspellings and deal with emoji are all key indicators of whether it is powered by AI. Because they can respond to a nearly limitless number of students at once, chat bots have the potential to provide real-time support at unprecedented scale -- which can streamline processes like admissions and enrollment and enable advisers to focus on students who need more hands-on, personalized guidance.
Natural language processing (NLP): The ability of a computer to parse accurate meaning from human conversation (or “natural language”). By using contextual clues, NLP can help machines make sense of what humans are trying to say -- for example, parsing the difference between trying to reach the accounting department and finding out the requirements for the accounting major.
Why it matters: Natural language processing isn’t new to higher education. In fact, admissions offices, beset by a torrent of questions when email became popular in the late ’90s, were among the first to use NLP to streamline operations -- and ensure rapid response to Gen X students who eschewed once-popular phones and call centers. Today, NLP can be the difference between a good user experience and a bad one -- just ask anyone who has been stuck on the phone with an automated system that can’t seem to send them to the right department.
Perhaps most importantly, NLP is getting better at learning the particular linguistic stylings of today’s students. Albeit nascent, advances in NLP hold the potential to parse almost any form of communication, meaning that virtual assistants or chat bots can understand a question even if it’s asked in a string of emoji.
In order to realize the promise of technology to help students navigate and complete their college education, it’s critical for institutional leaders to understand how that technology works -- and how they can use it to meet their students’ unique needs. Building a strong foundation in the language of emerging technologies should be any decision maker’s first step in exploring how these tools can fulfill the mission of all higher education institutions: to prepare students for a bright and successful future.