You have /5 articles left.
Sign up for a free account or log in.
There has been much concern in higher education about the possible negative effects of students using artificial intelligence for their academic work. For example, many professors worry that their students are using ChatGPT to complete assigned papers, instead of the students composing the assignments themselves. After all, ChatGPT constructs dinner party thank-you notes that are models of the genre, as one of us (AWL) has discovered to her great glee. Nevertheless, in contrast, there have also been higher education professionals who have embraced the use of AI to enhance student learning and success.
AI can indeed be useful to higher education in ways other than the writing of assigned papers. In this post we describe multiple ways that AI can be useful in helping students transfer from one college or university to another.
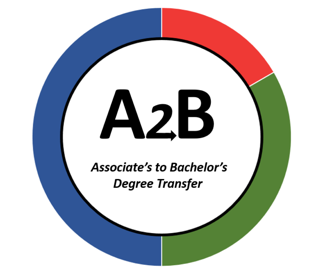
City University of New York
Nearly 40 percent of postsecondary students transfer at some point, and transfer students can face many challenges. A good illustration of these challenges involves the over 80 percent of community college freshmen who wish to obtain at least a bachelor’s degree, which necessitates transfer. Six years after entering community college, only about 11 percent of these students have received that degree. At least one of the reasons for this low success rate is the transfer students’ general education and major credits changing to elective credits upon transfer or disappearing entirely. Given that community colleges tend to have higher percentages of students from underrepresented groups, transfer impediments disproportionately harm students from those groups.
Thus, in addition to AI possibly saving institutions time and money, any assistance that AI can provide in facilitating transfer should be seriously considered for its potential to significantly increase equity in higher education.
So, how can AI help transfer? Let us count (just three of) the ways …
- AI can help identify likely course equivalencies.
Course equivalencies are the currency of the transfer process. When a transfer student submits a transcript with courses or other forms of credit that have not been previously evaluated, it can significantly slow down the transcript evaluation process. It can be a substantial amount of work for an evaluator to identify possible equivalencies, gather relevant materials and perform the analysis. As with any judgmental process involving multiple decision-makers, results can also be inconsistent.
By comparing available information on course descriptions, syllabi and prior-equivalency determinations, a natural language processing algorithm or large language model (types of AI) can identify likely course equivalencies in a variety of contexts. Such processes can be used to suggest course equivalencies to administrators or faculty who are reviewing a course or other form of credit for the first time, greatly reducing evaluation time and burden and supporting consistency in evaluation. A similar process could also be used to search a receiving institution’s catalog to identify additional or “better” equivalencies where an equivalency has already been recorded—the model may, for example, identify an equivalent course that would count toward a specific degree requirement when the previous equivalency was a general or disciplinary elective.
These two examples focus on a receiving institution’s catalog, but the model could also be focused externally—for example, searching online catalogs at other institutions to help identify potentially equivalent courses that a student could take on a nonmatriculated basis to meet a requirement.
Another example of applying the model externally would be to identify potential equivalencies at other institutions for a newly proposed course, helping to integrate transferability into the course design process. This might be particularly useful for colleges that are all within one system or state, where proposals for new courses could be required to include identification of equivalencies, thus heading off time-consuming, and possibly nonproductive, searches for course equivalencies after students have taken the new course and then transferred.
In all these cases in which existing equivalencies are inadequate, AI can help remove from students at least some of the burden of identifying better equivalencies, students who otherwise would have to appeal to faculty and administrators to change these equivalencies, actions that some students may be unlikely to take.
- AI can help students and those who support them understand and make use of program requirements and articulation agreements.
There is growing recognition that degree-requirement applicability—whether and how transferred courses count toward degree requirements at the destination—is the key question in students being able to carry their credits with them as they move from one institution to another. Unfortunately, determining applicability can be quite challenging. One reason is that program requirements are often quite complicated and are only recorded in natural language in a catalog. Articulation agreements, which are meant to mitigate the burden of this comparison exercise, ironically suffer from the same issue—they are typically rendered in natural, but exceedingly complex, language and recorded in a static format such as a PDF (which, even if they aren’t just stashed in someone’s dusty file cabinet, are rarely, if ever, consulted and/or are quickly out of date).
An AI model could be developed to process catalog information about program requirements and the courses that apply to them, as well as existing articulation agreements, and to render the information as structured data that could then be used in a more flexible way. For example, the structured data could populate an informational tool such as CUNY Transfer Explorer (T-Rex) that informs users about transfer credit applicability, to help potential transfer students plan. The data could also be utilized by faculty and administrators to compare and improve coherence of curricular offerings—either within an institution or in transfer pathways across institutions. Further, an AI model could use the structured data on program requirements as well as catalog and equivalency information to identify transfer options for individual transfer students or their advisers, based on the student’s existing records as well as their stated educational and career goals.
- AI can provide a first line of support to transfer students and other users of transfer information.
Access to knowledgeable transfer advising is critical to transfer student success, but due to high caseloads and the challenges of navigating complex information, far too many students don’t have such access. Administrators and faculty also may be stymied by a lack of accessible, authoritative information about transfer.
As is now commonplace in other situations, a well-trained AI chat bot can provide an effective first line of support for these users. It can understand natural language questions and respond in natural language. It can help direct users to existing resources that they might not discover on their own. And it can escalate the most complicated cases to (direct human) support, assisting advisers in triaging and prioritizing their caseloads.
What Underlies These Three AI Approaches?
In each of these scenarios, there are two core points that are essential to understanding and accepting the use of the technology.
First, AI, as employed to facilitate transfer, can be designed to utilize only authoritative catalog information, source data, policies and other information that has been written and approved by faculty and administrators, and it can therefore be limited to providing responses that are based solely on that information. AI’s responses regarding equivalencies, transfer pathways, etc., would then reflect an analysis of those input data, and only those input data. AI’s responses would not be created out of thin air and would consist of making time-efficient use of an institution’s own existing views. This is unlike the situation for ChatGPT, which can seem to “hallucinate” (i.e., can seem to generate responses that have nothing to do with its input) due to its having the whole of the internet at its disposal.
Second, at least initially, the AI output (for example: credit for course A should be given to a student transferring in with course B) can be treated as a recommendation to an institution as opposed to as a decision for the institution. The recommendation might become a decision failing sufficient evidence-based objections. However, until we gain experience with the use of AI for transfer, we humans should proceed with caution in implementing its output.
In this post we have described some of the great many ways in which AI can be helpful to students who wish to transfer, as well as faculty and administrators who support the transfer process. In the future, we will be using, within the Articulation of Credit Transfer (ACT) Project (under the Associate’s to Bachelor’s project umbrella) these methods and strategies to help ensure everyone has the information, guidance and best equivalencies needed for efficient and effective transfer, largely by means of T-Rex and its offspring. Our goal is to ensure that all students have the same opportunities to transfer and achieve their educational goals.