You have /5 articles left.
Sign up for a free account or log in.
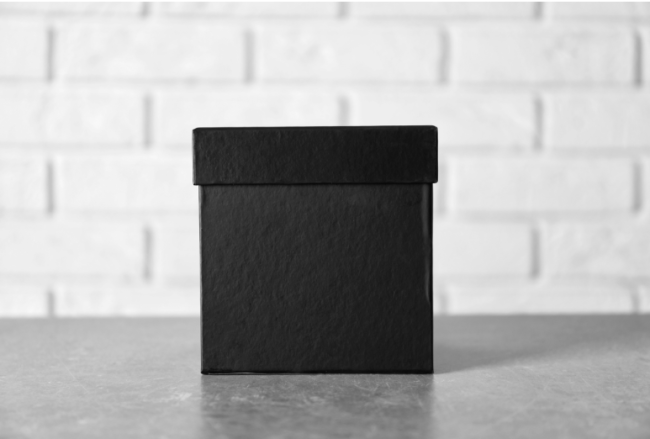
pixelshot
As someone who has not studied law, I will not attempt to explain the intricacies of the June 29 Supreme Court ruling rejecting Harvard and the University of North Carolina’s consideration of race in admission decisions. As a computer science researcher, though, I have found that computer science surprisingly has a lot to say about the importance of race in admissions.
We can view the college admissions procedure through the lens of an algorithm: high school students apply by inputting their grades, test scores and recommendation letters. These inputs are fed into an algorithm that then outputs a binary admission decision for each applicant—a one if the applicant is accepted and a zero if rejected. In this analogy, the goal of the algorithm designer is to make the admissions algorithm admit as many “well-qualified” students as possible—i.e., students who are most likely to do well in their classes if they were to enroll in that college.
Amid the debate on affirmative action, Jon Kleinberg and three co-authors performed a simple admissions simulation using nationally representative data on college students. They incorporated student data, including race, grades and test scores, from the National Education Longitudinal Study of 1988 to build several algorithms designed to maximize the number of students with a high college grade point average. The algorithms differ in how they use the various factors: most notably, the race-blind procedure does not have access to information about race, while the race-aware procedure uses race as a factor.
What they found is the race-aware procedure not only admits a more racially diverse class, it also admits fewer low-performing students—those students who would go on to earn low college GPAs—than a race-blind approach. The researchers investigate this difference and find that the higher inaccuracy of the race-blind procedure stems from different relationships of different predictors (such as high school test scores) and college grades by racial group. In other words, a single test score may correlate with different college GPAs for Black versus white students. “Only by giving the algorithm access to race can we account for this,” Kleinberg and his co-authors write.
These differences make sense, since standardized tests like the SAT don’t perfectly predict college GPAs. Different schools have different degrees of test preparation, and this is compounded by families who can afford to hire outside tutors for SAT preparation. As a result, significant racial and wealth gaps exist in standardized test scores, and a single test score isn’t a reliable indicator of a student’s capability. For example, a student might take the SAT for the first time without any preparation and score a 1400, while a student taking it for the third time with weekly SAT tutors might obtain the same score.
What this means is that an individual’s race, whether we like it or not, gives a signal about their background and lived experiences. This isn’t stereotyping; this is our reality, due to our nation’s historical roots in racism and oppression that legally ended only a generation ago. Its consequences persist to this day: segregated neighborhoods and school districts obtain highly localized funding, which results in an unequal distribution of resources across schools. Just like other aspects of our backgrounds, such as our genders, our hometowns or our incomes, paint a picture of who we are, race also provides more comprehensive insight into an applicant.
As an Asian American, I’ve had a growing interest in affirmative action, especially having been an undergraduate at Harvard while the Students for Fair Admissions case against the university was ongoing. I was curious about the decision process that went into my admittance, so I chose to view my own admissions file. I wasn’t expecting much, because the admissions game remained a mystery to me, but I do remember this: in my file, my interviewer had commented with the exact words, “not your typical math nerd.” I felt immediate confusion and disappointment; it was apparent that I stood out because I was compared to other Asian applicants. I thought that if I was of a different race, it would be a positive trait to be a “math nerd,” but instead I was judged against a stereotype. I wondered how many Asian students were rejected because their passion for academics was seen as “typical.”
You might therefore think I’d argue against affirmative action. But I also remember that the interviews were assigned the same day, and the only information my interviewer received was my name and the résumé I handed them, which didn’t mention race at all. Yet my race seems to have played a huge role in their impression of me.
My interview experience was therefore race blind on paper, but not in practice. In fact, this pretense of “colorblindness” is much more insidious than race-conscious admissions and will only worsen once colleges can no longer explicitly consider an applicant’s race. As Justice Sonia Sotomayor states, society “is not, and has never been, colorblind.” Preventing colleges from considering race will not stop the discrimination that students from marginalized groups experience on a daily basis. It will not stop admissions reviewers from making judgments based on the applicant’s race, and it will not stop teachers from writing more and less favorable recommendation letters because of their internal biases.
In fact, hiding race makes stereotyping even easier. People can and will implicitly assume an applicant’s race based on their name, as demonstrated in past research. This means that only the students who discuss their own race in their application essay will be able to accurately signal their race rather than have it be inferred in this manner. Students with stereotypical-sounding names will have even less control over how admissions committees interpret their identities.
Opponents of affirmative action may try to litigate these gray areas, but how much proof can they find when the admissions officers don’t have explicit access to information about race? I wouldn’t be surprised to see private universities become even more covert about their admissions processes in the future and get rid of any documentation—making admissions more analogous to the mysteries surrounding black-box algorithms.