You have /5 articles left.
Sign up for a free account or log in.
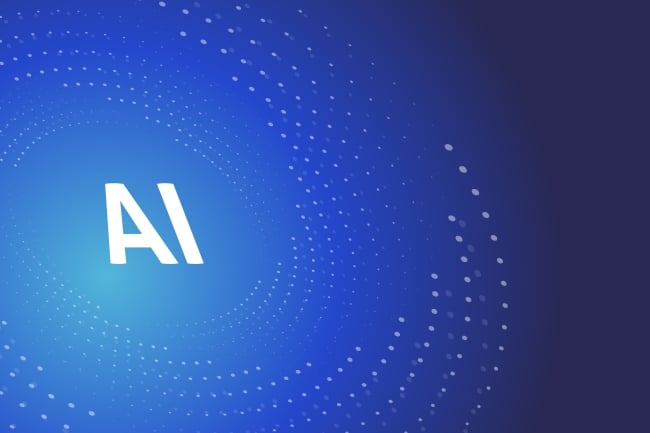
Sammy/iStock/Getty Images Plus
One of my favorite parts of teaching is welcoming students to the university in my introductory literature courses. As an educator, I think of myself as a host to the discipline, and I want to be an ethical and engaging host for new students who, I hope, will learn to love literature.
I started to think of myself as a host when I realized there is an unasked question that hangs in the room for students during these introductory lectures: Are you going to hurt me?
Students want to be inspired, they want to be exposed to new ideas and they want to make new connections. But they are also terrified that their professors may intentionally or unintentionally, directly or indirectly, do or say things that will hurt them.
This may be especially true for international students, who make up about 22 percent of the student body at my institution, the University of Manitoba. This past fall, as I joined other professors in welcoming new international students to the university, I wondered, what kind of learning community are we inviting them to join? Is it one where we can be sure that professors will not intentionally or unintentionally hurt them?
To welcome new international students at this moment is to bring them into a university system where we are undergoing a moral panic about the impacts of text-generating artificial intelligence programs like ChatGPT. About 43 percent of college and university students admit to using ChatGPT or similar AI applications, and about 50 percent of those who admit to using the tools say that they have used them to complete schoolwork. While the idea that so many students are openly cheating is alarming, these are self-reported numbers, and the likelihood is that the percentage is much higher. If we cannot get AI-based cheating under control, it could call into question the legitimacy of our credits and, in turn, the value of students’ degrees.
Yet university instructors are not trained on how to police AI-based academic misconduct, we do not discuss the potential biases we might bring to this policing work and we do not have systems to review our policing work to make sure we are doing a fair and equitable job. I did not become an educator to police students. I do not enjoy catching students who cheat or recommending punishments for them. Policing feels at odds with a pedagogy of hospitality, and like the inverse of what bell hooks calls teaching to transgress.
One of the many problems with asking professors to police, rather than educate, is that the rules around AI-based plagiarism are relative, opening up ample space for misunderstandings. The rules around what counts as cheating are in flux and can be different at different schools, for different faculties or departments within the same school, and even in different classes within the same department. Imagine being an international student coming to a new country, and perhaps learning a new language, while taking three different writing-intensive classes with three different professors who work in three different departments. You could have one instructor who permits some or all generative AI tools in their class, another who only allows the use of generative AI tools in specific instances or under specific circumstances, and a third instructor who will not allow students to use any generative AI, even tools found in Microsoft Word or Grammarly. These more restrictive policies, born of a tough-on-academic-misconduct mindset, may have unintended, but very real, consequences for students with accessibility concerns.
In short, we have professors acting as untrained police officers, defending an abstract ideal of academic integrity, combined with inconsistent rules around the use of AI across the university, subject to change from one faculty member or classroom to the next. What could go wrong?
A lot, I fear, especially for international students. Researchers at Stanford University have shown that AI-detection programs are biased against nonnative English speakers. In an article published in the data science journal Patterns, they found that while commonly used AI detectors “accurately classified the U.S. student essays,” they incorrectly flagged more than half (61.3 percent) of essays written by non-native-speaking students as AI generated.
Part of the reason for this is that AI detectors look at the complexity of the language in scoring papers. According to Stanford’s James Zou, the senior author of the above study, if the writing is more grammatically complex, uses a larger vocabulary and has more varied sentence structure, a detector is more likely to determine it was composed by a human. But, if a paper does not have lexical richness and grammatical complexity, then the detector is far more likely to assume that the writer was not human.
As use of AI (and AI detectors) increases, are university professors, intentionally or unintentionally, profiling international students, or those learning English as an additional language (EAL), for academic misconduct? The racial dynamic of this profiling is not at first obvious. We work with EAL students from Germany, Italy and France all the time, but those students do not seem to be the ones who are being investigated for academic misconduct. Rather, it tends to be students who are brown and Black who are disproportionately investigated for potential academic misconduct.
I would like to give raw numbers here to back up my point, but universities are resistant to openly tracking, or reporting on, instances of academic misconduct investigations by race or nationality, so we have no way of knowing how academic misconduct investigations may vary depending on skin color or citizenship status. Indeed, there is a complicated intersectional matrix to consider here in respect to how various elements of identity—among them citizenship and visa status, race, English language learner status, and markers of gender and class—can make accessing supports during an academic misconduct investigation more or less complicated. This intersectional matrix, moreover, can make it significantly more difficult to identify the potential sources of conscious or unconscious bias that graders and/or professors may have toward a student essay.
To guard against the real threat of conscious or unconscious bias impacting our international students, individual instructors must become mindful of the role conscious or unconscious racism may play in what papers they identify as potentially using AI and which students they accuse of academic misconduct. We also need larger, structural solutions. Universities need to track data over the next few years and provide clear reports outlining the demographics of those students accused of, and sanctioned for, academic misconduct. While each case of academic dishonesty is individual, when we start tracking these data in ways that account for the race and ethnicity of students and professors, we are likely to find some disturbing patterns. It is time for us to become better hosts to our international students by facing this problem and imagining ways to solve it that do not involve asking professors to do more policing.