You have /5 articles left.
Sign up for a free account or log in.
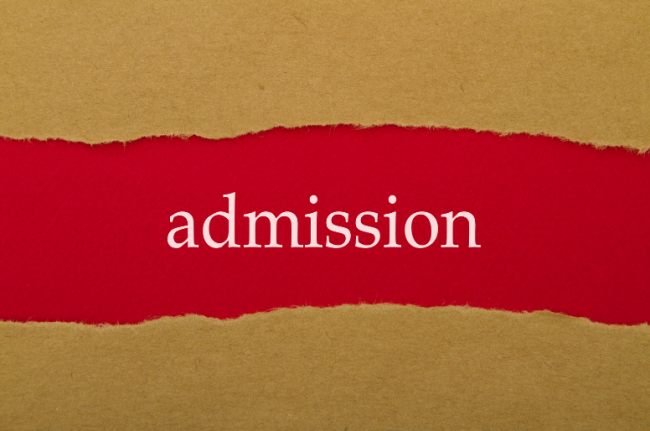
1001gece/Getty Images
Since the U.S. Supreme Court handed down its decision striking down race-conscious admissions practices in the Students for Fair Admissions cases (hereafter SFFA), colleges and universities across the country have been forced to revisit their admissions processes. This essay details how we approached developing a new admissions review process for Duke University’s master in interdisciplinary data science program four years ago and why we think the core feature of this new process—a mission-derived rubric that rewards a range of applicant attributes that are almost never all found in a single applicant—may serve as a model for ensuring a range of life experiences remain represented in the classroom in a post-SFFA world.
The Context
The Duke master in interdisciplinary data science (MIDS) program is a two-year applied data science master’s degree program that aims to matriculate about 50 students a year, selected from about 900 applicants. The program was started in fall 2018, but I will focus on our experiences since a change in program personnel forced a redesign of our admissions process in fall 2021. Since that time, the program has matriculated 130 students across three cohorts. (Attentive readers will notice that 130 divided by three does not equal 50. The program struggled with yield and melt prediction during the pandemic, resulting in two cohorts that were below our target.)
The 130 matriculated students come from 25 different countries, including four in sub-Saharan Africa. As undergraduates, they majored in everything from statistics and math to geological engineering, cognitive science, psychology and public policy. They range in age from 21 to over 40, and more than half identify as women. Seventeen have master’s degrees in other fields: one has an M.B.A., one student is simultaneously completing a neuroscience Ph.D. and four have postgraduate medical degrees.
While enrolling a class with such a wide range of life experiences is not necessarily exceptional in a pre-SFFA world, as detailed below we have both theoretical and empirical reason to believe that the vast majority of this variation—variation we feel has been critical to the success of our program—is the result of a SFFA-compliant approach to application review.
A Multifaceted Rubric Based on Mission
We began development of our new admissions rubric by gathering our faculty to determine the attributes we most wanted in our students to achieve our program’s mission of training effective, impactful and ethical data scientists. As a program, we felt strongly that this required training not just in technical methods, but also in critical thinking, communication and the ability to work effectively in teams across a range of personal and cultural backgrounds.
To that end, we concluded our prototypical ideal MIDS student would be someone who had excelled academically in a field of their choosing, gone into the world to put their education to use and discovered they needed to improve their technical training to accomplish their goals. Imagining this ideal student helped us to agree on five distinct attributes we were seeking in admitted students:
- A demonstrated ability to excel academically—for our program, we further decided that applicants must demonstrate an ability to do quantitatively or mathematically rigorous work, but they need not have majored in statistics, mathematics, data science, computer science or a similar field;
- A mature perspective regarding the promise and limitations of data science and a thought-out, clearly articulated reason for pursuing a degree in data science;
- Data science–relevant life experiences that would contribute to the educational experience of other students in the program;
- Nonacademic work experience;
- An understanding of the importance of interpersonal skills in data science and a demonstrated ability to work in teams.
While these attributes were developed in large part by imagining our ideal student, in practice we have found the magic of this rubric is that almost no one gets top marks in all five categories. Rather, the diversity of attributes evaluated in the rubric gives rise to a pool of high-ranking applicants with extremely diverse profiles—we certainly have many students who are coming straight out of undergraduate institutions with great grades in math or statistics, but we also have 30-something professionals who did not excel as undergraduates but who have since matured and now want to acquire new skills to be more impactful in their careers.
This differentiates our rubric substantially from others I have seen used by other graduate programs that score applicants along a relatively unidimensional “good-bad” spectrum, either by prioritizing a relatively narrow, strongly correlated set of attributes, or by using rubrics that score components of application packages—statements of purpose, letters of recommendation, etc.—from bad to good.
To be clear, this model is not without downsides. Chief among them, we matriculate students with very diverse backgrounds when it comes to their exposure to data science, computer science and statistics, something that causes quite a few pedagogical challenges. As a program, we’ve had to repeatedly adapt our curriculum to ensure all incoming students are well supported, regardless of background. For example, we created asynchronous, online mini review courses in statistics, linear algebra and basic Python that students complete the summer before they arrive; we have students get to campus three weeks before classes start for an in-person, mandatory programming boot camp to help onboard those who arrive without prior programming experience (and break more than a few bad habits among those students who are more experienced programmers).
In their first semester, about two-thirds of our students (those with less prior programming experience) also take a course designed to provide them with explicit training in a range of skills students who grew up playing with computers know but that are almost never taught explicitly in statistics or computer science courses; we have to be very careful with messaging to prevent issues of impostor syndrome arising from interpersonal comparisons early in the program.
However, the educational and pedagogical benefits of having such an interesting range of experiences in the cohort more than offset the challenges. Our older students often have to lean on their younger cohort mates for help on more technical assignments, but they more than repay the favor by modeling time management and other soft skills. And it’s hard to overstate the contributions this range of backgrounds brings to classroom discussion—when we discuss topics like communication with nontechnical audiences, students with industry experience are able to share their own professional experiences. Discussions of the ethical issues surrounding data science are significantly improved—I rather doubt the realness of the ethical issues surrounding Google’s collaboration with the Department of Defense to improve drone targeting would have sunk in for students nearly so well had the class not included both active-duty U.S. military personnel and Pakistani students.
Our Rubric Post-SFFA
The third attribute in this rubric—whether an applicant has unique data science–relevant life experiences —is clearly the category that is most potentially problematic post-SFFA. However, there are two reasons to believe that even in a post-SFFA era, a revised version of our rubric will still allow us to matriculate cohorts with the wide range of life experiences we have come to treasure. The first is that our concept of data science–relevant life experiences has always been interpreted broadly. High-scoring applicants in this category have included a public health worker, a radiologist, a fish biologist, veterans, active-duty military personnel, a Ministry of Transportation employee from a developing country and a fine arts student turned museum curator. To the extent to which race, ethnicity or nation of origin contributed to this category, it was only one of many contributing factors, most of which are unaffected by the SFFA ruling.
The second is that using data from our fall 2023 applicants, we can examine how candidate rankings would change if we remove that entire rubric category from consideration. We find that of the 321 candidates for whom we have complete ratings—essentially the top third of the 900 applications we receive every year—excluding the entire diversity of life experience category would only have moved 17 students of the 157 who were actually admitted below the threshold we used to determine admission.
Of course, this exercise isn’t a perfect approximation for how we expect our rubric to perform post-SFFA. For one, this rubric category takes into account both dimensions of diversity that were and were not identified as problematic by SFFA (e.g., this rubric category gives credit for unusual backgrounds like studying neuroscience or working for government agencies). As such, excluding this category in its entirety is quite conservative. Nevertheless, this analysis certainly corroborates our qualitative sense of how the rubric operates.
The Supreme Court decision in the SFFA case has unquestionably changed the landscape for college and university admissions. As admissions chairs across the country work to revise their rubrics and review processes, I hope that the experience of our program may help others as we all do our best to achieve our educational goals in an SFFA-compliant manner.