You have /5 articles left.
Sign up for a free account or log in.
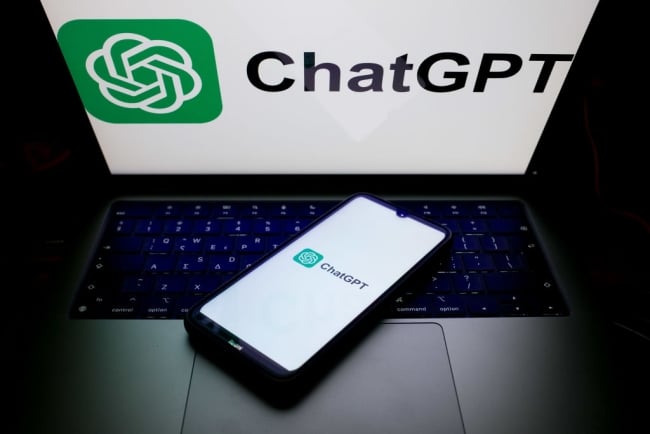
NurPhoto/Getty Images
A survey of about 3,800 postdoctoral scholars conducted by Nature showed that 17 percent of postdocs use chatbots daily and 43 percent use them weekly for such tasks as “refining text” (63 percent), “code generation/editing/troubleshooting” (56 percent), and “finding/summarizing the literature” (29 percent). The relatively high percentage of researchers using chatbots for finding and summarizing the literature is both surprising and concerning, given that hallucinated/fake citations generated by ChatGPT made many headlines and have been discussed extensively in public media and the academic literature.
ChatGPT produces fake but realistic-looking citations, because it is not connected to a database of scholarly publications. As the acronym GPT (Generative Pre-trained Transformer) suggests, it is pretrained on large amounts of textual data, the scope of which has not been officially disclosed beyond vague descriptions released by their developers such as “vast amounts of data from the internet.” While human researchers (ideally) read and then cite a previously published paper, ChatGPT produces citations by processing text data and generating a highly probable response to a request, in this case, for a citation. A similar issue has been observed when using ChatGPT in mathematical calculations, where a highly probable response can be outright incorrect.
To warn users, ChatGPT developers have included a disclaimer underneath the input box that reads “ChatGPT can make mistakes. Consider checking important information.” Furthermore, paid users of ChatGPT can use context-specific GPTs that offer add-on features on top of the vanilla version of ChatGPT to conduct specific tasks. The introduction of the GPT Store has made available numerous new custom-built GPTs (developed by third parties) that use the ChatGPT application programming interface (API) for various tasks. Examples include GPTs that solve math and algebra equations (e.g., Math Solver, Linear Algebra Solver), teach physics in ways that match different learning styles and age groups (e.g., Physics Tutor), and help users with cooking (e.g., Recipe Generator: Cooking Assistant).
There are also various GPTs (e.g., Consensus, Scholar GPT, Research Papers, Scholar AI) that offer scholarly citations in response to a query statement (e.g., “coffee is good for human health”). These GPTs resolve the issue of hallucinated and fake citations and offer citations that are bibliometrically correct. However, this convenience comes with a hefty price. Since these GPTs use the ChatGPT API, they inherit most of its limitations. In addition to our lack of understanding about how exactly they work (the black box problem), the lack of reproducibility, debatable reliability and prevalence of biases suggest that these GPTs enable a shoddy research culture, resulting in at least four distinct ethical issues.
- Unreliable and nonreplicable searches. Substantial financial investment and a wealth of expertise have gone into developing robust, orderly and well-maintained scholarly indices, which enable reliable and reproducible searches. For example, according to the National Institutes of Health’s National Library of Medicine, PubMed registers “retraction and erratum notices, expressions of concern, corrected and republished articles, comments, duplicate publications, updates, patient summaries, and republished articles” to ensure that researchers find all the necessary information associated with a citation. However, nonreproducible GPTs that have not been vetted by field experts and librarians may not have access to all available sources, and may not keep an up-to-date record of retractions, errata and expressions of concern. Accordingly, they could produce unreliable and nonreplicable searches, which negatively affect the integrity, accuracy and veracity of the research record.
- Increased likelihood of citation ethics violations. It is currently unclear to what extent citations generated by GPTs are accurate, as there has not yet been a systematic evaluation of these tools. Since researchers are ultimately responsible and accountable for all decisions made throughout the research process, allowing GPTs to search through the scholarly corpus and offer a handful of sources that support a claim stands to negatively impact the accuracy and integrity of citations. Engaging with previously published material in a responsible manner is a complicated and consequential matter, the very bedrock upon which researchers find gaps in the literature and develop and test new hypotheses. Moreover, as recent allegations of misconduct against top university officials in the U.S. have shown, the consequences of irresponsible use of the literature could come back to bite researchers at any level, many years after a research publication.
- Increased likelihood of bias in the literature. While scholarly indices present the indexed corpus of abstracts and a rapidly growing corpus of full-text articles that match keywords or a search string, GPTs provide researchers with a select list of available sources. Furthermore, indices like PubMed have specific filters and user guides that are frequently updated and maintained, but GPTs may come with no filters and no instructions on how to use them or how to avoid mistakes. Accordingly, biases in algorithms and input statements could result in citations that fail to support claims made in a publication, do not reflect available nuances, or, worse, do real harm by offering citation support for unsubstantiated and inaccurate claims. Unless researchers thoroughly read and validate every offered citation before use, these GPTs could propagate inaccurate and slanted information about the published record, increasing misinformation and pseudoscience far into the future.
- Enabling shoddy research. Since these GPTs allow researchers to use unstructured statements and sentences as a search term (instead of leveraging structured keywords or the Medical Subject Headings thesaurus, for example), they enable indolent researchers to cite publications based on their hunches without any understanding of the literature, or even without reading an article’s abstract. Consequently, frequent and unfettered use of these GPTs may permanently impact the scholarly method, impacting the ability to identify and access evidenced-based research and advance discovery through rigorous inquiry.
Use of ChatGPT and other available chatbots can help make a wide range of research tasks more efficient; however, care must be taken to ensure responsible use. While these specialized GPTs address the issue of hallucinated and fake citations, they create additional ethical issues with detrimental consequences. Due to the breakneck speed of their development and adoption (GPT Store reports that Consensus has been used more than 5 million times, and Scholar GPT more than 2 million times), these GPTs have not been tested for accuracy and reliability, and researchers have not been trained on their responsible use.
To appropriately address these gaps, we need further assessment of these tools’ veracity, the development of guidelines and best practices for their ethical use, and meaningful training for researchers. Ultimately, a range of interventions are required to prevent GPTs from spreading misinformation, pseudoscience and biased views that will undermine norms of research and ultimately erode trust in science.