You have /5 articles left.
Sign up for a free account or log in.
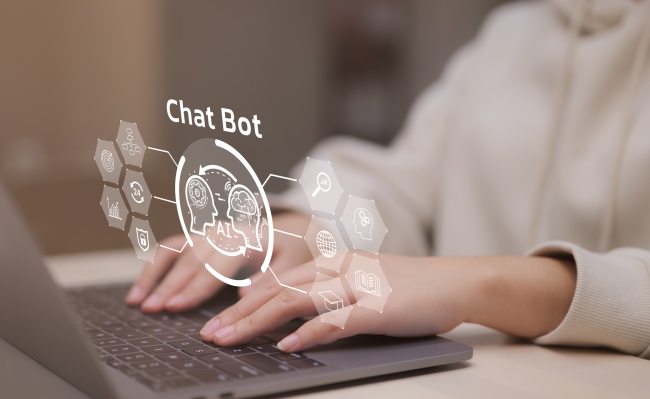
Supatman/iStock/Getty Images Plus
Academia is abuzz about the impact of generative artificial intelligence on student learning. Opinions are abundant, but without credible and relevant markers of what’s going on, it’s hard to discern who has an accurate read or what constitutes good advice.
Recent online national surveys, such as Tyton Partners’ “Time for Class 2024,” provide a 30,000-foot read on how generative AI is disrupting the landscape of higher education. But most academics don’t work on that national landscape—just in one corner of it. They need to know what’s happening in their particular corners: universities, divisions, departments.
In April and early May, students and I studied one specific corner: a small Christian liberal arts college in the Midwest. Student researchers administered a survey to more than 400 undergraduate students in 24 randomly selected face-to-face courses, stratified by academic division. Their pitch to students was simple: fill out a one-page anonymous survey and immediately receive a full-size candy bar. The response rate was 83 percent, with most nonresponders simply absent from class. For context, this homegrown survey nearly doubled the sample size (and more than doubled the response rate) of another national survey. Our sample size reduces the margin of error to around plus or minus 2 percent—enough to inform practical pedagogical decisions.
For a wider audience, a high-quality snapshot of a particular institution offers a complement to national surveys. Readers can develop some sense of what’s happening at their own college or university by triangulating among these different sources. But even if our findings are not generalizable, our approach might be: The survey was simple, even vintage. It would be easy to replicate and tweak for the needs of other institutions. More broadly, whatever the long-term impact of generative AI on higher education, it’s hard to imagine a direction forward that doesn’t in some way track how students and faculty use generative AI at the institutional level. Data at the institutional level empowers administrators, faculty and students to discuss and make informed decisions about teaching and learning.
Here’s a brief summary of our findings and an initial step in that direction: In our sample, 66 percent of students reported using generative AI for assignments in the course where they filled out their survey. Usage rates notched up to 75 percent in the social sciences, with the humanities and natural sciences at 63 percent and 55 percent, respectively. These numbers echo high adoption rates reported by Tyton Partners; they found 59 percent of college students use generative AI for schoolwork at least monthly. Our estimates are also notably higher than the 37 percent reported by an Intelligent.com survey in February.
This top line has practical consequences. Some discussions of the future of higher education seem to assume a 100 percent adoption rate by students. That may be where things are headed; however, that rate is not the current reality. As of this spring, a credible starting point for instructors is to assume most but not all students will use generative AI in their course. A “most but not all” heuristic conveys the urgency of addressing generative AI in course expectations and instructional design. It suggests a critical mass of students may be prepared to discuss the ethical and practical nuances of generative AI in an academic setting, while a substantial minority may need a basic introduction to the technology already embedded in their devices.
Generative AI enters into students’ study habits in different ways. About half of students reported using generative AI to get feedback on or develop their own ideas. That’s just a little more than the 45 percent who used it “to better understand material I read or studied.” Other strategies placed bots more squarely in the academic driver’s seat: About one of three students used generative AI “to get by when I don’t have much time” or “to summarize material so I don’t have to study.” One in six indicated they have used generative AI “to write paragraphs of assignments for me” in their respective courses. Clearly, these strategies have varied implications for students’ learning processes and outcomes.
We wanted to know what instructors would think of students’ responses, so we surveyed full-time faculty at a facultywide meeting. All or nearly all who were present participated, for a sample of 57 of about 80 full-time faculty at the institution. Comparing their responses yields some of our most helpful findings and suggests the contours for future conversations.
First, faculty thought students use generative AI more often than students said they did. For example, we asked instructors how often they think a typical student uses generative AI for daily assignments in their courses. Instructors’ most common response was “about half the time.” But 85 percent of students indicated they “never” or “very rarely” use generative AI for daily assignments. Fewer than 10 percent reported “about half the time” or a higher rate of usage. Of course, it could be that students gave inaccurate responses on the survey. It may be that the collective commotion or ambiguity around AI-generated work leads faculty to perceive it even where it doesn’t exist. Probably, this finding reflects a mix of both.
Second, our findings suggest the most common ways students use generative AI are also the ways faculty—at least on average—found most acceptable. About eight in 10 faculty, for example, said it was at least sometimes OK to use generative AI “to better understand what I read or studied,” a strategy about half of students reported using in their course. Optimistically, one could interpret this alignment to mean that—at least for right now—students’ norms and practices aren’t that far off from instructors’. However, when students were asked directly if their use of generative AI was ethical, most expressed modest agreement, even if they had done things most instructors disapprove of—things like “writing paragraphs of assignments for me” or “summarizing material so I don’t have to study.” So the alignment might not reflect consensus so much as risk mitigation: Students might often use generative AI in ways that are unlikely to draw instructors’ disapproval, or at least that seem defensible if instructors do push back.
Finally, our findings indicate a need to clarify expectations regarding use of generative AI. Sixty-three percent of students agreed that their faculty member has set clear expectations for generative AI use. Interestingly, only about half of faculty said the same for their own courses. One basic and measurable objective is to increase both of those percentages and understand how they vary in relationship to one another.
This summer, I have been sharing the survey findings with various departments and divisions, with hope that the ensuing discussions gain pedagogical traction this fall. Of course, the empirical value of these findings ticks down as students and generative AI rapidly evolve. And it falls again when the findings migrate to another institution. But even findings from a particular time stamp and corner of academia have value in a market where evidence is scarce.
Looking ahead, evidence about generative AI usage doesn’t have to be scarce. Scientific evidence is, after all, what most academics produce. The most helpful knowledge will be particular—collapsed in space and time—so academic leaders and instructors understand generative AI practices in their respective divisions, departments and courses. It’s not hard to imagine colleges and universities formalizing that sort of data collection through institutional research. That task is only one of the myriad novel questions confronting institutional research offices.
In the meantime, new forms of data analysis are at students’ fingertips, fueling every variety of speculation; this survey’s data provides a glimpse of what those fingertips are actually doing.