You have /5 articles left.
Sign up for a free account or log in.
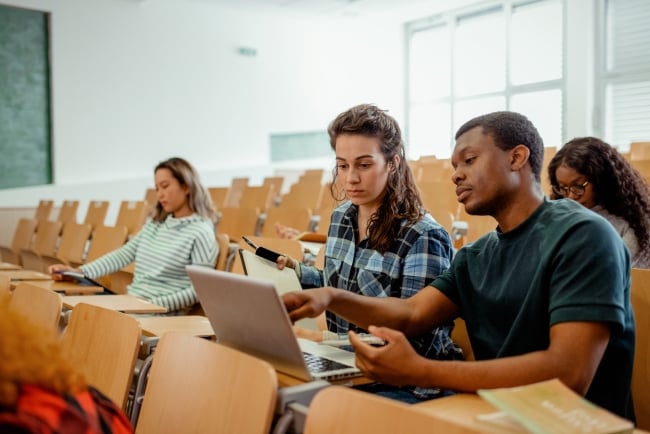
A new tool from the University of Texas Austin supports student learning on course topics including those who need additional help with the material or who want to challenge themselves and learn new, fringe topics.
PixelsEffect/E+/Getty Images
Over the past two years, generative AI has blazed its way on to college campuses, first in students’ hands and increasingly in the hands of administrators and instructors to improve campus operations and enrollment management as well as teaching and learning.
One of the greatest challenges of using generative AI in teaching is providing students with skills without interrupting the process of learning or introducing errors or misinformation. The University of Texas at Austin is in the initial stages of launching a custom GPT model, UT Sage, which will serve as a tutor of sorts for students who need help related to a specific course.
In this episode of Voices of Student Success, Julie Schell, assistant vice provost of academic technology at UT Austin, shares the inspiration behind the tool, her work with AI in the classroom and teaching the ethics of AI use.
An edited version of the podcast appears below.
Inside Higher Ed: How did you get started in the AI world? When did you start studying that?
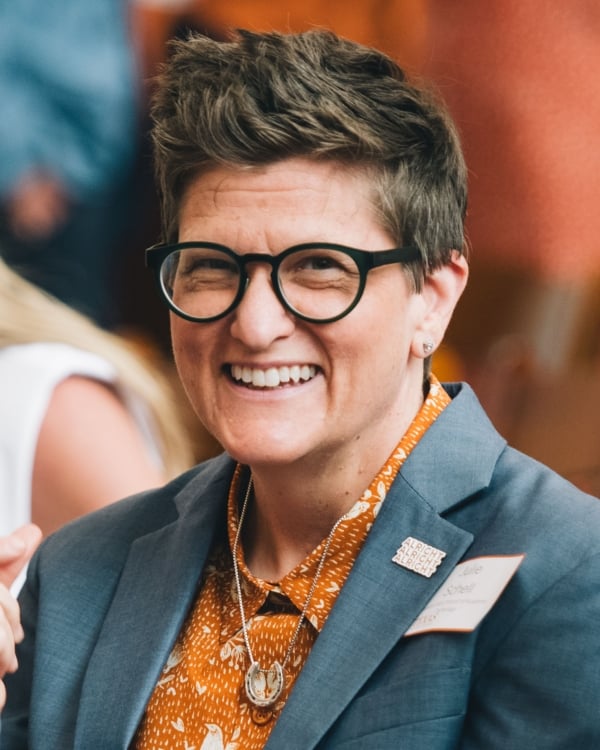
Julie Schell, assistant vice provost of academic technology at the University of Texas, Austin
Julie Schell
Julie Schell: I teach a class called design pedagogy. And I had been working on AI starting in about November 2022, with the launch of ChatGPT, and things on campus had started to ramp up around that.
I’d been working on some generative AI conversations and policies and thinking about how we’re going to respond to the generative AI craze here at UT Austin for about six months, and then things got really real in the summer of 2023 because I had to teach my own class in the fall of 2023.
I know that AI is really important in design, and I felt I would be doing my students a real disservice if I didn’t know what I was talking about. I spent about 20 hours teaching myself how to use Midjourney and code in Discord to develop generative AI images with Midjourney and really understand it for myself and do some real deep dives into it—so that in the fall semester of 2023, I was prepared to help my students navigate these, what I think are pretty ambiguous waters.
I would say that I had both contexts where I was working on it from a policy level as an administrator, but then for myself, I felt I owed it to my students to understand what it is, how to use it and what they needed to know about it connected to our own learning outcomes.
Inside Higher Ed: I think that’s been the challenge for faculty members, where it seems like AI can circumvent work for students, and it can be a great supplement to help them in their careers and lives after college. But it can also take shortcuts in the learning and teaching process. In your work, and how you have been thinking about AI, where are you sort of seeing those two themes of AI use in the classroom being weighed and discussed?
Schell: Look, AI is full of paradoxes. One of the really interesting things about AI from a teaching and learning perspective is that it can teach you almost anything, right, but it can also teach you bad information and it can teach you wrong things. It can speed up your work and really help you be faster at producing work, but it can also make you look really sloppy. It can help you feel more creative, but it can also make you sound like everybody else.
So I think it’s really important to recognize these paradoxes and talk about them with students, and really articulate clearly what is the learning outcome for this particular class or this particular teaching concept that we’re working on, and what are ways in which using AI could foster learning of that topic, and what are ways in which it could inhibit or negate learning of that topic, and why might you not want to do that?
I think it’s really important as a faculty member, as a teacher, to be clear on that myself, and then to articulate to that to my students and help them become the architects of their own ethical blueprints about how they’re going to use AI or not.
Inside Higher Ed: I love that, “architects of their own ethical AI blueprints,” because ultimately what we’re trying to empower students, in all facets of higher ed, to do is to learn and take those tools to make their own decisions. We’re not trying to make carbon copies of students or faculty [members].
But helping them on that journey is really hard, especially like you mentioned, you have to educate yourself on these issues so you can be an informed expert, or at least have some sort of knowledge about these issues. And that can be a challenge as the tools evolve and grow and the landscape of AI has become so complex as well.
Schell: When that semester started, I had really clear AI policy in my syllabus, and I talked with students about this policy on the first day of class.
In our one of our first projects, students have to design user personas. In the syllabus, and in my conversation, I talked with them about how to cite generative AI use in our class. And this is dependent—a lot of people ask me, how do you cite generative AI use? It depends on the discipline, but in design, there are some precedents, and so I taught them those precedents.
So the policy was, you can use generative AI as long as you cite it. I got one of my student’s user personas, and it had a really well-designed image that really helped me get the feel and have empathy for the particular user. And there was no citation, so I gave student the feedback about how effective I thought it was and well-designed it was, and the student just said, “Oh, I use generative AI to make that.”
I was really shocked. I was surprised because we had talked about it, and we had gone through, and we had done demonstrations on how to do the citation. I realized it wasn’t as if the student was trying to hide that they had used generative AI, and in that moment, I just realized that the ethical muscle that people have around using generative AI, it doesn’t have as much strength and resilience as their ethical muscle around plagiarism.
I realized it wasn’t as if the student was trying to hide that they had used generative AI, and in that moment, I just realized that the ethical muscle that people have around using generative AI, it doesn’t have as much strength and resilience as their ethical muscle around plagiarism.”
—Julie Schell, assistant vice provost of academic technology at UT Austin
Look, all of my students know not to plagiarize. You know not to plagiarize. I know not to plagiarize. Sure, some people still do it. Some students still do it, but we know when we take someone else’s work and we try to pass it off as our own, that is academic misconduct and that is plagiarism. We have a really built-up muscle for that that we’ve developed over our lifetimes.
But with generative AI, I don’t think that ethical muscle is built up in the same ways. And so I believe, as a teacher, it is my job to see academic integrity as a process and to approach that with curiosity and to ask, why was that still confusing? What can I do to help that student understand, where did things go wrong? And we had a conversation about it, and it worked out really well.
If you look at things that students are saying right now, you’ll see that 70 percent really do want to have AI in their classes as part of their lessons, and as part of the instruction that they’re receiving. But what they care about is learning how to engage with the ethics of AI. I don’t think they need to know how to use the practical aspects of AI, but they want to know how to use it responsibly and ethically.
For me, that was in a really important moment, and it’s shaped what I’m doing, both as a teacher, but as well as what I’m doing as a person responsible for guidance around AI implementation and teaching and learning here at UT Austin.
Inside Higher Ed: I think that’s a good transition into sort of the larger institutional efforts that you all are making around AI. So you have this new tool, UT Sage, that you all implemented. Can you give us the story behind that? Where did this come from?
Schell: As you’ve probably been able to tell, I like to experiment a lot, and I really like to try to learn something that I’ve never been exposed to. I try to teach it to myself first, because I’m interested in how people learn complex things, right?
So when the custom GPTs became available, within the second that they were available for public use, I built a custom GPT. I built the custom GPT to do something that I teach in my class, which is how to write a good learning outcome. When I saw what it produced, I literally like, shot back from my desk, and I thought, “This is going to change everything.”
I immediately sent the GPT to my boss, and he was like, “OK, we’re onto something here.” So from that moment, we started thinking about how we could empower other educators across the university to develop these custom tutors to help students work through— We’re focused on topics that [students] often struggle with or that they find difficult.
From that, Sage was born, and I can walk you through a little bit more about what Sage does and how Sage is unique, and not just a regular custom GPT that you might find on one of the various tools that are available.
Inside Higher Ed: Yeah, please tell us more.
Schell: I think I’ll start with, thinking about you had asked the question about, what are ways that generative AI might further learning, and what are ways that it might negate it.
One of the things that we think that generative AI might be really good at is helping address what we often see in classrooms which are part prior knowledge gaps. When you come into a class, there’s a couple things that determine whether you’re going to be successful in that class or not, and one of the central predictors is the quality of your prior knowledge.
Oftentimes, when students come into a class, they will enter that class either with missing prior knowledge or they’ll enter that class with incorrect prior knowledge. Those are both gaps. At the same time, you might also have students that come into your class that have an abundance of prior knowledge, and they’re far more advanced than other students.
When you [the instructor] come into that class, you face this challenge, and, as an educator, you have to teach at the middle or a little bit to the left [of the knowledge curve]. In order to address this spectrum of prior knowledge, you kind of have to teach a little bit to the left of that curve, so that you cater to everyone. But then the students are at the very tail end of both get a little bit left behind.
So one of the things that we are thinking about with Sage is, are there ways in which Sage could potentially help students, particularly those students who have these prior knowledge gaps, and maybe aren’t as confident to come into office hours or ask questions. Maybe they still find—because the gap is so wide—when they get a response to the question, they’re still confused. Are there ways that we could engage them and help them strengthen that prior knowledge using generative AI, and are there ways that we could help students?
We have a faculty member who’s using Sage whose students really want to learn fringe topics, but the faculty member doesn’t have time to teach the students the fringe topics, so they’re working on a tutor to help students learn fringe topics. You can kind of see both of those spectrums.
What we’re doing with Sage on the student side is trying to find a way to engage students in developing and addressing some of these prior knowledge gaps in a way that meshes with how people learn.
When a student logs into Sage, what they’re going to see is a typical text-based chat bot interchange. We’ve got a tutor right now on a topic, logistic regression. The student comes in, the faculty member has preprompted some starter questions that the student could click on, depending on where they’re at. So it could be, “What is logistic regression?” Or it could be, “I’m really good at logistic regression. Give me some really tough questions that I could practice with.” So the student has some autonomy where they can choose on which of those that they want to pick, or they can type in a prompt themselves.
One of the things that we’ve programmed Sage to do in response, so if the student says, “What is logistic regression?” is to be more Socratic in the responses. Not just to give a definition and leave it at that, but to start engaging the student in some that powerful Socratic questioning.
For example, if you ask Sage, “What is logistic regression?” Sage might reply with a definition, but then say, “Have you ever needed to predict the outcome of an event?” And then let the student answer that. And the student might say, “Yeah, I’ve wanted to predict the outcome of a race,” for example, or a sporting event. And then what Sage would do—say [the student] says soccer. Sage will actually walk them through a soccer example that’s relevant to them and help understand logistic regression in the context of that particular example, which is great. Because one of the things we know about prior knowledge is that learning something new, it really benefits when you can connect into something that you already know, and Sage is functioning in that fashion from the student side of the chat bot.
Inside Higher Ed: That’s super cool. You talked about this a little bit earlier, but sometimes generative AI tools can feed students misinformation if they’re searching for things. How have you and other faculty members tried to add those barriers to make sure that Sage is only providing accurate and helpful information to the student and not hallucinating or sort of going off the rails?
Schell: One of the very first things that we’ve done is, we have a responsible AI and teaching and learning framework that we operate from. That framework involves these big six limitations of using generative AI.
The first thing you see when you when you enter into Sage as a student, is you see a disclaimer that AI makes mistakes and that there are important limitations to AI, and you can click on a link that will direct you to this thing that we call the big six limitations.
That’s one way; transparency is the key, and to always remind people that there are limitations, not just hallucinations, but there are other limitations that we want people to be aware of in order to engage responsibly.
But the reality is that we actually think that generative AI does not pose the challenge of our students’ lifetime, but I actually think that dealing with ambiguity does pose the challenge of our students’ lifetime. It’s not just AI where they might get bad information, they might encounter implicit bias, they might encounter security and privacy concerns. They might encounter misalignment, which is different than a hallucination—it’s when the AI gives you something that you didn’t ask for.
So we think it’s really important to teach students to evaluate and critique the output, and regardless of whether you’re using the top model or you’re using a model that maybe has more difficulty with those kinds of things is to always be critiquing and evaluating the output. And not just of generative AI, but anything that they come across, being really discerning critics and not just adopting that wholesale.
This is a concept in education called keeping the human in the loop. We train students to make sure that there’s always a human in the loop, not just on the input, but also on the output.
Inside Higher Ed: Not every student or every faculty member at every institution trusts AI yet. Have you had conversations with your students or with your colleagues about how to make— We talked about you can’t trust AI completely—but helping them feel like AI can be a copartner in this work?
Schell: I definitely think there’s a spectrum of readiness for using generative AI in institutions, among students and faculty, and I think it’s really important to meet people where they’re at.
I actually think it’s pretty healthy to not have full trust in generative AI … It means that you’re actually at a higher level of readiness. We call this being AI forward and being AI responsible. We want people to use the tools, but we want to use them in a way that has clear awareness of these big six limitations.
But one of the best things that you can do is to experiment. Whether you’re an AI skeptic, you’re anti-AI, you’re AI agnostic, you’re AI forward, you’re AI experimental, the best thing to do is to use generative AI for at least 15 to 20 hours.
I’ll tell you, when you start to do that, you will have an awareness. The first hour or so, I’m sure you experienced this yourself, Ashley, but when you first started using it, I know when I first started using it, it’s so seductive. I was at a family event, and I was so enthralled with using Midjourney to produce images that I basically told my family, “I’ve got a work call that I have to do, so I’m going to be a couple hours,” and I just went into the back room, and I just kept producing images and using Midjourney to do this, because it’s so seductive and so amazing.
But after about 15 to 20 hours, you should come to a huge insight, which is that—and this is what I want every student and every faculty member to know, but really the students—it is not better than you. It might be faster, it might have access to a bigger knowledge base, but it doesn’t have your experience. It doesn’t have your voice, and it is not you. And when I share that with my students, I find that they are able to make the really effective, ethical decisions, responsible decisions about their use. I think that’s what’s the most important thing when you’re talking with someone who is really not trusting it.
Inside Higher Ed: What kind of feedback have you heard from students using UT Sage?
Schell: One of the really cool things about UT Sage is that, it’s still in proof of concept right now. One of the things that we’ve been doing is that we operate from a human-centered design framework, so we believe the customer is always right.
Sage is in closed beta right now, which means that it’s a small set of faculty and a small set of students [using the tool], and I got to be the first person to test Sage with my students on a topic that we were studying. It was really exciting to see, they thought there was a lot of potential, but they had a ton of feedback about things that weren’t working.
One of the things we got feedback on was that it was too Socratic, so it just wasn’t answering any questions that they were asking. That feedback helps us tune, like, we don’t want the direct answer, but this is a little bit too much Socratic questioning.
Inside Higher Ed: I can imagine Sage being like, two plus two is four, but what do you feel about this number? Like, how does this make you feel?
Schell: Exactly.
The other thing that we found during this test is that there was an accessibility problem. One of the students couldn’t read something, due to an accessibility issue in Sage. So we got feedback on that, and we’re able to fix it.
We’ve gotten really good feedback from the students in making improvements to Sage, and we’ve gotten feedback about the potential that something like Sage could offer.
The sort of killer app around Sage is not necessarily the student-facing part of it. That’s important, too. But if you’ve ever built a custom GPT, you’ll know that the tool will ask you a series of questions to help you build that custom GPT.
We’ve actually programmed Sage to ask the faculty member a set of questions that are aligned with everything we know about effective teaching. So Sage is also, in addition to being an AI tutor, an instructional designer, a virtual instructional designer for the faculty member that focuses on learner-centered pedagogy.
The first thing Sage will ask is, “OK, what’s the topic?” Because we need to know the topic to get the model going. But then the next questions that Sage will ask is “Who are your learners? What do they need? What are the common challenges or difficulties that they face with this particular topic?” Then Sage will ask, “How do you help them get through those difficulties or those struggles? What are some of the tricks of the trade that you’ve built up over your career as a faculty member to help students move through those misconceptions?”
That’s the first question. The second question Sage will ask [is] “What do you want students to know? What do you want them to be able to do, and what attitudes do you want them to have about this particular content?” And then Sage will ask, “how are you going to assess? So how should I know whether the student is able to do those things?”
Then finally, Sage will ask for resources, so a faculty member could upload lecture notes, or they could upload a reading. We’re working on being able to upload other types of media in the future. I think that is something that’s really special about Sage. It operates as a virtual instructional designer that’s baked in key principles of learner centered pedagogy.
Inside Higher Ed: It’s almost like you’re training Sage to be a TA and to know sort of what the faculty knows and how they teach.
Schell: That’s a great analogy, because just like any TA, you know, Sage isn’t going to have the same knowledge as the faculty member is going to have, but they’re going to be custom-trained by the faculty member themselves.
Inside Higher Ed: When it comes to future goals with teaching with generative AI or learning alongside generative AI, where do you see things moving? Or what are your hopes?
Schell: My real hope is that we get a real firm understanding about what responsible AI use is for teaching and learning.
I think we need to be really transparent and clear about how to use generative AI in ways that protect our students’ privacy, that protects their security, that protects their intellectual property rights, and also encourage and support academic integrity. I think that once we get that responsible AI framework for teaching and learning in place and solid at our institutions, then I think we can move to a place where we’re using generative AI to further learning, like filling prior knowledge gaps.
Another thing that generative AI can be really good for is helping you engage in metacognition. A lot of people think metacognition is just thinking about your thinking, but it’s way more than that. Metacognition is really gaining awareness of your learning state. If the topic is logistic regression, prompting you to try to take a moment to figure out where you’re at, like, when it comes to logistic regression, are you at a zero or are you at a 10? Well, I’ll tell you, I’m like at a one right now on logistic regression.
Inside Higher Ed: I’m a negative five, personally.
Schell: Yeah, right, exactly. If we can use generative AI to do things that we know from 100 years that are like these really potent ingredients in learning science, and we can get that into a tool like Sage or other generative AI tools that can promote these really effective behaviors, like engaging in metacognition.
Because what happens next, after you become aware of your learning state, that you will then self-direct, most students will then self-direct or self-regulate their learning, which is really the Holy Grail. If you can self-direct and self-regulate your learning, then you’re going to be a successful learner.
I think that the future, for me, in using a tool like Sage or generative AI is really tuning it to be aligned with the ways in which it meshes with how people learn, rather in rather than in the ways in which it could negate how people learn. And I think that’s a personal and disciplinary dependent decision.
Inside Higher Ed: I know Sage is in the beta state right now, but the data that Sage can use both to inform its own work and how it’s engaging with students, but also faculty members in real time and in the real-world experience, if you will. Have you all considered how that data can both inform teaching practices but also inform Sage?
Schell: Definitely. So one of the things that we’d love to do—this is a feature request, and maybe by saying it now, the tech team will really want to do it.
One of the things we’d really like to do is be able to give the instructor a heat map or report of the areas in which the students are finding confusion, or they’re particularly finding difficult … so that the faculty member can spend time during class working on those more complex needs with the students face-to-face. That’s a wish list [item] that we have for Sage.
The other wish list that we have for Sage is to be able to tune based on student response. For example, using the student’s responses, let’s say that you have a student that’s asking a question about logistic regression. They keep asking for examples. And Sage gives example one, and then the student says, “I don’t really understand that. Can you give me another example?” And the student says, “Well, is it like this?” Can Sage turn to start to be more empathic when the student is demonstrating that they’re struggling and showing a lot of confusion?
And on the other side of the coin, if the student is really getting it and can tackle anything that Sage throws at it, can Sage potentially become more challenging? And I don’t mean from a personality standpoint, I mean from a topic standpoint, like a challenging instructor and giving the student those harder tasks to be able to achieve.
I think using the input that the students deliver, those are two of the things that we’re thinking about wanting to do.
Inside Higher Ed: It seems like it would be a little complex to code, but would be super engaging and exciting for the student to be able to sort of see those pathways Sage diverges on.
Schell: Definitely, and then nobody gets left behind. I think that’s what’s really exciting about it.
Inside Higher Ed: This is something, I think often when we talk about these generative AI tools or accessible resources, we’re thinking about the student that’s struggling on their own. But I also love the idea that it can be for the overachiever who wants to just keep digging into [content] and really has enthusiasm for it. They don’t need to find that on their own. They can use these tools to continue learning, because that’s what we want, is for our learners to keep learning.
Schell: One of the things that I always coach my students on—and actually I do have a couple generative AI lesson plans that I’ve developed and that I use—is I always use a mixed-method approach.
For example, in my class, students have to write a statement of teaching philosophy, but they’ve never heard of what that is. They don’t know what that is. They’ve never seen one before. It’s kind of confusing. What’s the difference between a statement of teaching philosophy and a cover letter? You know, it can get confusing.
One of the things that I have students do is use generative AI to help start build up some of that prior knowledge, but then actually do primary research and secondary research that they would normally do with respectable resources, and then compare and contrast to develop that really sticky knowledge that I want them to have. I think for students who are really advanced, they will naturally take that advanced, mixed-methods approach. And we want to encourage all students to do that.
Listen to previous episodes of Voices of Student Success here.